Update (May 30th, 11PM): Two more domains (A10 and B12) will be expanding by 2 seats each shortly.
Update (May 27th, 10PM): One new domain (A17) will be added, and three domains (A09, A16, and B02) will be expanded, for a total of 10 added seats.
Welcome! đź‘‹ This page was just updated for Fall 2024. Read the information at the top of the page, then scroll down to see information about each domain.
Domain Descriptions
DSC Capstone, 2024-25 @ UC San Diego
Overview
Welcome to the capstone program! The capstone program is a two-quarter sequence (Fall 2024 and Winter 2025) in which you will be mentored by a faculty member or industry expert in their domain of expertise. By the end of Quarter 2, you will design and execute a project from that domain in teams of 2-4. You can see the projects from last year at dsc-capstone.org/showcase-24.
At a high level, here’s how the capstone program is organized:
- In Quarter 1 (DSC 180A), you gain background information in your mentor’s domain, by means of replicating a known result. By the end of Quarter 1, you will have completed a replication project (known as the “Quarter 1 Project”) and will have a proposal for a more independent project (known as the “Quarter 2 Project”, or the capstone project).
- In Quarter 2 (DSC 180B), you execute the Quarter 2 Project you proposed at the end of Quarter 1.
You can see the syllabus for last year’s capstone offering here.
Enrollment
First pass for enrollment will begin on Friday, May 24th. The available domains are not listed on the Schedule of Classes; instead, they are detailed below. Most domains are run by UCSD faculty, but some are run by industry partners (denoted with an Industry Partner badge).
Use the information here to choose the domain you’d like to enroll in. Once you’ve chosen a domain, all you need to do is enroll in the corresponding discussion section for DSC 180A once first pass comes, space permitting. (Nothing is stopping you from waiting until second pass, but it’s less likely you’ll get a domain of your choice.) Note that you cannot change domains between DSC 180A and DSC 180B.
All of the information here – domain offerings, section times, descriptions, summer tasks, etc. – is subject to change as mentors provide us with more information.
As of this writing (Wednesday, May 22nd at 1PM), the section sizes in the Schedule of Classes haven’t yet been updated. Trust the information here, not there.
How should I choose a domain?
You should aim to choose a domain that suits your interests and preparation. By clicking the Read more button underneath a domain, you’ll get to learn more about the mentor, their mentoring style, the prerequisites that they’d like their students to have, tasks that they’d like their students to work on over the summer, and their students’ capstone projects in previous years (if any).
âś… Good reasons to choose a domain:
- You are sufficiently prepared.
- You find the topic interesting and would be motivated to study it.
- You are likely to work well with the mentor given their mentoring style and research background.
❌ Bad reasons to choose a domain:
- The mentor has good teaching evaluations.
- The industry partner is a big company.
- The section has space for you and your friends at a time that you like.
Everything you produce for the capstone will have to be public on the internet for the rest of eternity with you and your mentor’s names attached to it – you want your capstone work to be something that you’re proud of and can talk about on job and graduate school applications. Who do you want writing you a recommendation letter?
What happens in DSC 180A?
In addition to meeting with your mentor each week, there will also be methodology instruction delivered by the capstone coordinator and the methodology course staff. However, the majority of this instruction will occur asynchronously, in the form of readings (like this one). This means that you can mostly ignore the lecture and lab times that appear for DSC 180A on the Schedule of Classes. A few of the lecture slots may be used for the capstone coordinator’s office hours or for one-off guest lectures, but we don’t plan to use the majority of the times.
All prerequisites for DSC 180A will be strictly enforced. The prerequisites for DSC 180A can be found here. If you took either DSC 140A, DSC 140B, or DSC 148 to satisfy the machine learning prerequisite, you may need to submit an Enrollment Authorization System request in order to enroll in DSC 180A in fall quarter.
Note that since DSC 180A and DSC 180B are both 4-unit courses, you should expect to spend 12 hours a week on capstone-related work each quarter. Plan your class schedule accordingly – try not to take several time-consuming classes alongside the capstone.
Who is overseeing the capstone?
With any questions about the capstone sequence itself, feel free to email Suraj Rampure (rampure@ucsd.edu) for now. Suraj is leaving UCSD but a new capstone coordinator has yet to be identified; once it’s clear who will be in charge of the capstone moving forward, this page will update with their contact information.
With any questions about the content of a particular domain, contact the mentor. With any questions about enrollment, please contact Student Affairs in the VAC.
Filter by subject area:
đź’Š Medicine and Bioinformatics
🤝 Fairness and Causal Inference
đź§ Theoretical Foundations
🗣️ Language Models
⚙️ Applied Data Science
đź’Š Medicine and Bioinformatics
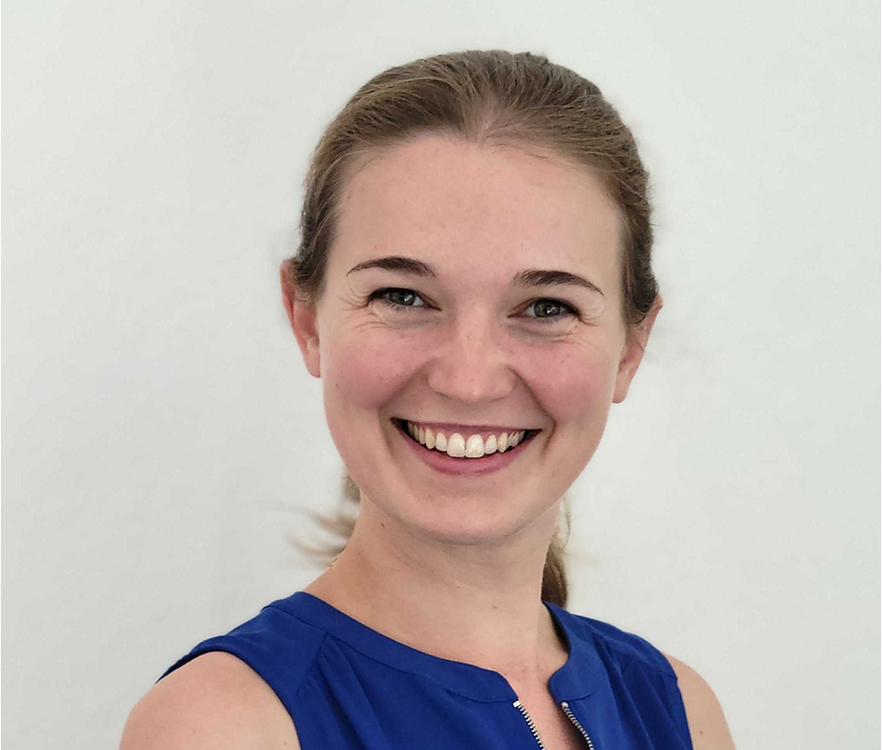
Precision Genomics with Personalized Genetic Risk Prediction
Tiffany Amariuta • tamariutabartell@ucsd.edu
A01 8 seats Wednesday 11AM-12PM, In-Person
A polygenic risk score (PRS) is a weighted sum of an individual’s risk alleles across one’s genome for a particular phenotype, i.e. disease or other measurement. The weights are typically the effect sizes of the risk allele, estimated by a genome-wide association study (in the case of complex traits / polygenic diseases) or an eQTL study (in the case of gene expression). PRS have great potential to revolutionize preventive care. In theory, an individual may arrive at the clinic not knowing their genetic susceptibility to a disease, have their DNA sequenced, and learn what is their lifetime risk for the disease. There is a theoretical liability threshold of PRS at which individuals who have a PRS value lower than the threshold will not develop the disease and those with a value higher than the threshold will develop disease. For diseases with a monogenic basis, it has been shown that the same degree of disease risk can be conferred by polygenic risk alone (Khera 2018 Nature Genetics). PRS are generally useful for understanding how predictive genetics is of disease and how disperse the genetic contributions are. PRS is especially useful in understanding genetic liability when individual effects are too small to be easily detected by genome-wide association studies (Purcell 2009 Nature). In this capstone, students will use population genetics and genomics data to assess individual risk for disease outcomes and transcriptomic measurements. Students will learn to work with genotype data from 1000Genomes and genetic association data from genome-wide association studies (GWAS) and transcriptome-wide association studies (TWAS).
Read more
- About: Before starting her lab in San Diego, Tiffany earned a B.S. in Biological Engineering at MIT and went on to conduct graduate research with Dr. Soumya Raychaudhuri as part of the Bioinformatics and Integrative Genomics PhD program at Harvard Medical School, where she studied the genetic susceptibility of autoimmune diseases and other polygenic diseases. During graduate school, Tiffany developed machine learning methods to predict the functionality of regulatory variants, which had applications to transcription factor binding prediction, eQTL mapping, heritability enrichment analysis, and trans-ancestry portability of polygenic risk scores. She pursued post-doctoral research studying tissue-mediated genetic effects with Dr. Alkes Price at the Harvard School of Public Health. Now, Tiffany is an Assistant Professor in the Halıcıoğlu Data Science Institute and the Department of Medicine at the University of California San Diego. In her free time, Tiffany enjoys figure skating, hiking, tennis, beach volleyball, and spending time with her dog, Dax.
- Mentoring Style: I run the capstone independently, without help from grad students or postdocs in my own lab. I like to meet with everyone simultaneously and lead discussion sections each week about the papers/data we are looking at and analyses students are doing. I am hands on in the sense that the weekly tasks are predetermined rather than being abstract; this changes in the second quarter where students will follow their own research plan (with my input and guidance).
- Suggested Prerequisites: Bioinformatics, gene expression (RNA-seq) data, genotyping data, computational biology, genomics
- Summer Tasks: Summer reading: Study that generated data we will use: https://www.ncbi.nlm.nih.gov/pmc/articles/PMC3918453/ Polygenic risk scores: https://www.ncbi.nlm.nih.gov/pmc/articles/PMC3912837/, https://www.ncbi.nlm.nih.gov/pmc/articles/PMC6128408/ Privacy concerns regarding genomic data: https://www.nature.com/articles/s41576-022-00455-y Genetic effects on gene expression: https://pubmed.ncbi.nlm.nih.gov/32913098/
- Previous Project
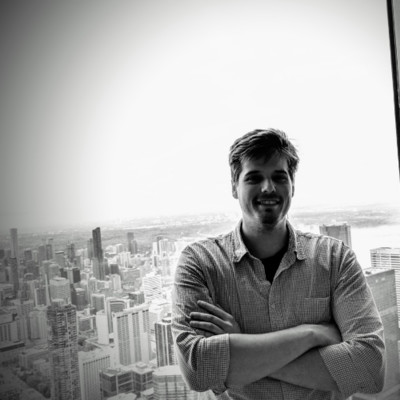
Sepsis: Using Clinical Healthcare Data Science to Identify and Combat an Infectious Killer
Kyle Shannon • kshannon@ucsd.edu
A02 4 seats Thursday 1:30-2:30PM, In-Person
Students will explore the world of inpatient ICU care by examining severe infection management and detection using the MIMIC dataset, a comprehensive, publicly available database of de-identified ICU patient data. This project will familiarize participants with healthcare data nuances and the critical role EHRs (Electronic Health Records) play in clinical decision-making. Through this experience, students will gain insights into the broader context of clinical decision-making and public health, learning to leverage EHRs and clinical data science for developing potential products, reports, and or health policies. They will better understand the US healthcare system, ICU operations, and the decision-making process for complex infectious cases like sepsis. By studying the work of multidisciplinary teams, students will gain a deeper understanding of intricate ICU cases and the patients' journeys through this challenging healthcare landscape. Additionally, they will appreciate the complexities of conducting data science in a demanding environment.
Read more
- About: Hi 👋 I’m Kyle Shannon, as a professional in the public health and data science fields, I am dedicated to improving healthcare accessibility and enhancing patient outcomes, particularly in rural America. My journey began at UCSD, where I studied in the CogSci department as an undergraduate and discovered my passion for Data Science when it was still an emerging field (2012). I later pursued my master's degree in Data Science at UCSD, and eventually co-founded a startup focused on healthcare access in rural America. My enthusiasm lies in data science projects that directly impact patient health outcomes, and I maintain a keen interest in cognitive neuroscience and CNN ML systems in healthcare (my masters' thesis). Outside of work, you can find me on a tennis court or shrouded in the ambiance of a cozy cafe while tackling projects... preferably while it is raining.
- Mentoring Style: My goal is to create a capstone experience that emulates a practical job setting, guiding students in effectively interacting with managers and data science leads, asking relevant questions, and fulfilling their responsibilities. I may assume various roles (e.g., DS lead, stakeholder, hospital admin, manager) to offer diverse perspectives. I incorporate a business angle to discuss the project's broader context, encouraging students to envision their work in scenarios such as product development or hospital consultancy. This approach helps them grasp real-world applications and develop a compelling narrative for their projects. I prioritize accessibility for my students throughout the week, for example, via Discord, and may involve domain experts for them to interview and learn from professionals in ICUs and EHR data. This context adds valuable insight and humanizes the data/system. I often hold informal meetings with my students over coffee to discuss progress and answer questions. Occasionally, I expect them to provide progress reports and mini-presentations, simulating a real-world organizational experience. Let’s have some fun while hacking away at healthcare! But also learn the grave responsibility that comes with working in such a high stakes setting.
- Suggested Prerequisites: These are not prerequisites, but are classes that might be helpful if you have taken them previously:
- BILD 26. Human Physiology (4)
- USP 143. The US Health-Care System (4)
- FMPH 101. Epidemiology (4)
- FMPH 102. Biostatistics in Public Health (4)
- BICD 140. Immunology (4)
- BIEB 152. Evolution of Infectious Diseases (4)
- Summer Tasks: The following are recommended summer domain readings and tasks. Getting through some or all of these, especially if you are a bit unfamiliar with the domain, would be a good idea. And help you to hit the ground running in the fall. I will be available during the summer to meet with you as a group once or twice if you wish.
On my capstone website, all material from last year is available, and I have put a note by the items I think would be good candidates to begin with over the summer: For clarity, during the summer, the three areas I recommend focusing on would be:
- Familiarizing yourself with EHR data
- Learning about the MIMIC dataset
- Beginning to understand a bit more about clinical critical care in an ICU
- Previous Project
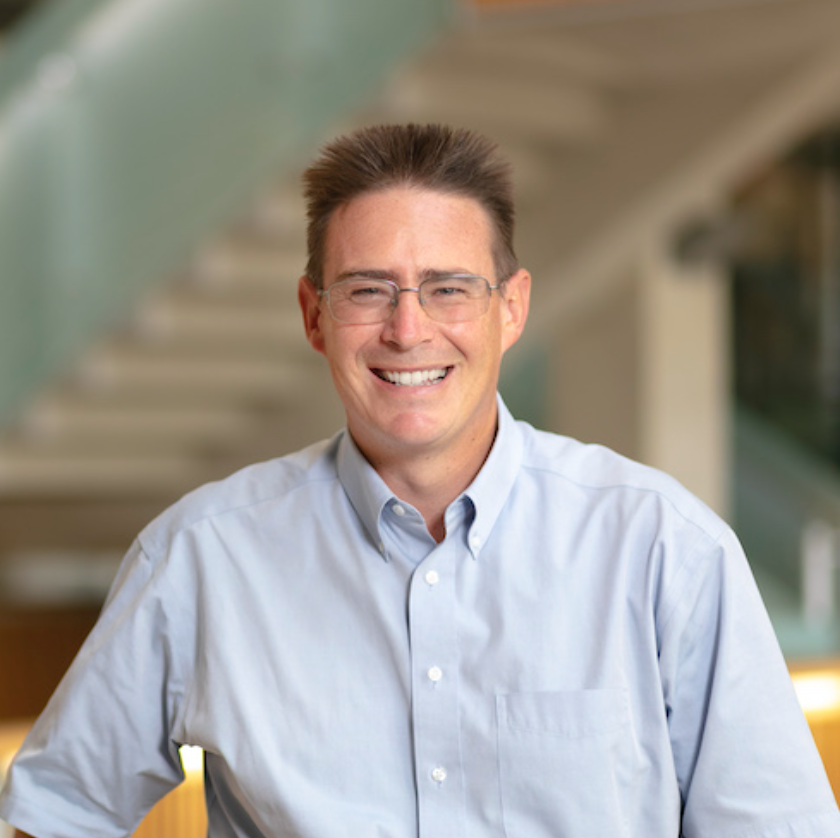
Applying Transformer Models to Microbiome Data to Improve Human and Environmental Health
Rob Knight • rknight@ucsd.edu
A03 8 seats Wednesday 3-4PM, In-Person
Did you know that more than half the cells in your body are bacteria, and at least 99% of the genes in your body are not from the human genome? We are discovering how the microbiome, all the microbes that inhabit us, are linked to different health conditions, ranging from inflammatory bowel disease to Alzheimer's disease and depression. We have been exploring different applications of transformer models to improve classification and regression of microbiome data with respect to patient or environmental variables, to identify novel antimicrobial agents (last year's project), to relate the microbiome to other data layers such as the metabolome or to brain imaging, and to incorporate the biomedical literature into systems that can make microbiome data accessible to physicians, patients, and citizen-scientists. We will explore the current space around the microbiome, pick a recent paper or preprint to replicate results from, and then explore a new iteration of the method or apply the method to a new dataset. Our microbiome analysis platform Qiita contains data from over half a million samples, so there are many potential questions to explore that could have a high impact.
Read more
- About: I grew up in New Zealand, and was always interested in biology and computers from a young age. I did a BSc in Biochemistry at the University of Otago in New Zealand, a PhD in Ecology and Evolutionary Biology at Princeton, and postdoctoral work at the University of Colorado. At UCSD, I am a Professor in 4 departments - HDSI, CSE, Bioeng, and Pediatrics, and I direct the Center for Microbiome Innovation. My highly interdisciplinary lab pioneers techniques for microbiome specimen analysis and data analysis, including in the Human Microbiome Project, the Earth Microbiome Project, and the American Gut Project/Microsetta.
- Mentoring Style: Class will be assisted by graduate students and/or research staff in my lab who are experts in the domain and/or deep learning techniques to be applied. The first few weeks will be spent assessing what techniques this year's students are most excited about learning or applying, and refining a specific application area (e.g. a particular algorithm or disease or both). More independence typically leads to a more interesting project, but given the state of the field the ability to replicate results from a cutting edge paper and apply it to a new dataset that we have access to is perfectly acceptable. Prior knowledge of the microbiome, or even biology, is not expected - the goal is to learn something new!
- Suggested Prerequisites: None
- Summer Tasks: Most recent projects have used PyTorch so familiarity with that is a good idea. My TED talk, although it pre-dates our use of AI, gives a sense of what we do and what the key questions are. https://www.ted.com/talks/rob_knight_how_our_microbes_make_us_who_we_are?language=en
- Previous Project
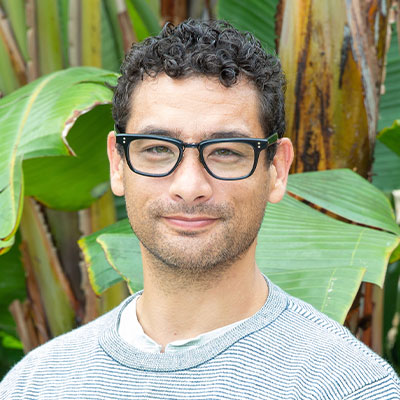
Hierarchical Latent Variable Models for Neural Data Analysis
Mikio Aoi • maoi@ucsd.edu
A04 6 seats Tuesday 10-11AM, In-Person
Recent years have seen an explosion in the ability to routinely record from hundreds of neurons simultaneously. Data analysis methods, however, have not kept pace and there are many scenarios in which structured latent variable models could provide effective and interpretable data summaries. in these capstone projects we will review the relevant neuroscience problem setting and neurophysiology, review the history of dimensionality reduction in systems neuroscience, and learn the mathematics of latent variable models. We will then develop some novel models that could be utilized in current neuroscience experiments.
Read more
- About: I'm a computational neuroscientist with a research focus on data science methods for neuroscience experiments. My academic career was anything but linear. I started my undergraduate career with no interest in math and knowing nothing about neuroscience and now I teach and do reach at the intersection of both. I'm interested in closely examining the way that we ask questions using data and dreaming up new ways of extracting meaning about what we are and how brains function from the raw numbers.
- Mentoring Style: I'll provide a great deal of context, coaching, and direction for the project but my capstone students will need to spend time figuring things out for them selves. We'll start out slow with exercises and "home works" that will be challenging but will prepare students with the requisite skills and to think critically about their work and take on challenges as they come.
- Suggested Prerequisites: Probabilistic modeling, optimization, and linear algebra.
- Summer Tasks: Please read Chris Bishop's Pattern Recognition and Machine learning. Specifically: Chapter 2, especially section 3 and the summary table on page 93, Chapter 3, Chapter 12, Chapter 10, and Chapter 6
- Previous Project
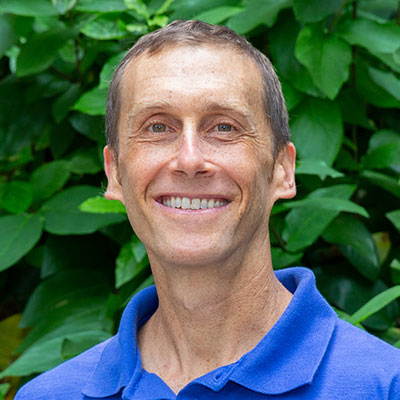
Multivariate Prediction from Human Brain Functional MRI Data
Armin Schwartzman • armins@ucsd.edu
A05 6 seats Wednesday 3:30-4:30PM, In-Person
Multivariate prediction from functional MRI is a technique for understanding how brain activation patterns map to thoughts or experiences, akin to mind reading. Instead of analyzing brain regions in isolation, this approach considers how joint patterns of activity distributed across the brain can predict psychological or clinical outcomes. In this capstone project, we will learn how to 1) work with neuroimaging data in Python, 2) select informative features, and 3) train models using supervised machine learning. We will focus on interpretability and think about how the model parameters might help us better understand the relationship between brain structure and function.
Read more
- About: With an undergraduate degree in electrical engineering, I discovered statistics for my PhD and have been doing data science since then (even when it wasn't called by that name). Much of my work involves signal and image analysis, but I'm interested in many theoretical and applied problems, even philosophical. Outside of academia, I like doing music, dancing, swimming, surfing, and more.
- Mentoring Style: Mentoring will involve data science PhD student Gabriel Riegner. Students are expected to take ownership over the project. This implies taking initiative in learning about the topic (from the assigned material and other sources), implementing the methods in code, being resourceful when needing help, and asking questions. Students are expected to put in their best effort, plan their time over the quarter, make substantial progress each week, report on it each week, and come up with an action plan for the next steps (as opposed to waiting for the mentor to give instructions). In other words, be independent and ask for help when needed.
- Suggested Prerequisites: Probability and Statistics (e.g. CSE 103, ECE 109, MATH 180A, MATH 183, MATH 189)
- Summer Tasks: To start, we will replicate the results from the Haxby paper that introduced this method: Distributed and Overlapping Representations of Faces and Objects in Ventral Temporal Cortex (link: http://graphics.cs.cmu.edu/courses/16-899A/2014_spring/thevisualworld/HaxbyPietrini2001Science.pdf). Reading material:
- Background on Functional MRI: Chapters 2, 5, 9, 10 from Handbook of Functional MRI Data Analysis (link: https://www.cs.mtsu.edu/~xyang/fMRIHandBook.pdf)
- Multivariate Prediction Tutorial (link: https://dartbrains.org/content/Multivariate_Prediction.html)
- Introduction to Nilearn Tutorials (link: https://nilearn.github.io/stable/auto_examples/00_tutorials/index.html)
- Nilearn Tutorial on Replicating the Haxby Result (link: https://nilearn.github.io/stable/auto_examples/02_decoding/plot_haxby_different_estimators.html#sphx-glr-auto-examples-02-decoding-plot-haxby-different-estimators-py)
- Previous Project
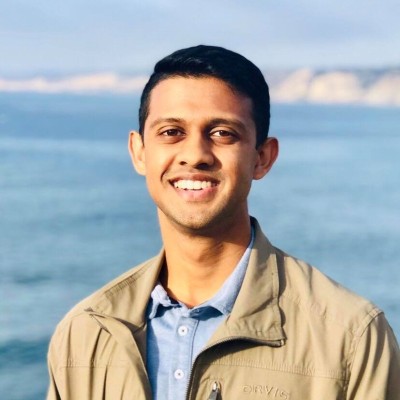
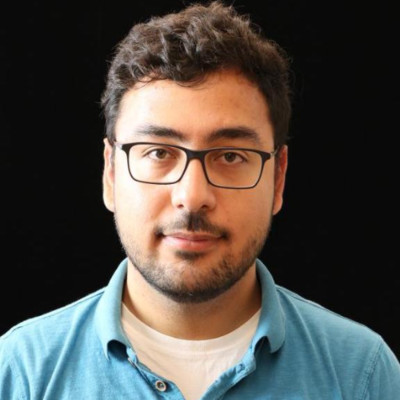
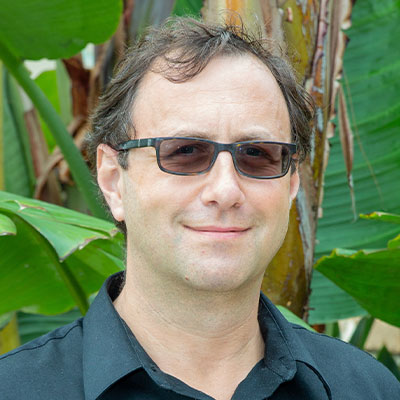
Graph Attention Networks in Genomics
Utkrisht Rajkumar, Thiago Mosqueiro, and Misha Belkin • utkrisht96@gmail.com
and thiago.mosqueiro@gmail.com
A06 8 seats Monday Evening, Hybrid
Graph Neural Networks (GNNs) are the next frontier in machine learning, designed to navigate the intricate web of connections in real-world data. They harness neural networks to unravel hidden patterns and insights buried within complex relationships, from social media graphs to molecular structures. GNNs are already making waves in fields like biology and drug discovery, transforming phenomena into graph structures to predict protein interactions and uncover new drug candidates. As the field evolves, we're exploring exciting frontiers like Graph Large Language Models, bringing the power of language models to graph-structured data. The primary objectives of this project (1) to comprehend biological complexities through the lens of machine learning and (2) to design and apply tailored GNN models to address intricate biological problems.
Read more
- About: Utkrisht is an Applied Scientist at Amazon.com since 2022, specializing in Graph Machine Learning, NLP, and Computer Vision to detect high-velocity fraud events and cybersecurity threats in Amazon and AWS. Some of his innovations include web tracking of buyers and sellers in Amazon, generating billion-scale graphs of Amazon traffic, and spam protection for Amazon customers. Utkrisht is a San Diego local and completed his undergraduate degree (2017), master's degree (2019), and PhD (2022) all at UCSD. He also has a mini-MBA from the Rady School of Management, UCSD. During his PhD, Utkrisht conducted research on applying deep learning techniques to discover elusive mutations in cancer genomes. He has been a teaching assistant for courses at both undergraduate and graduate levels in the Computer Science and Bioengineering Departments. Outside of work, Utkrisht spends his time flying (he is a private pilot) and playing pickleball.
Thiago is a Sr. Applied Scientist at Amazon.com since 2018, working on a variety of topics such as large language models, recommendation systems, neural networks, de-biasing methodologies, causal inference, and ML Ops. Originally from Brazil, Thiago is a physicist by training, finishing his PhD on 2015 on mathematical modeling of biological neural networks. Since then, Thiago worked as a Postdoctoral fellow for UCSD on projects involving ML applied to neuroscience, systems biology, and finance. Thiago also taugh two graduate classes on big data for finance as visiting professor for the Rady School of Management, UCSD. In 2017, Thiago moved to UCLA where he was a Postdoctoral fellow and part of the Collaboratory where he created a 3-day intensive course on Machine Learning for biologists which continues until today. Outside work, Thiago spends most of his time playing music. - Mentoring Style: For the first quarter, we will meet as one group to gain familiarity and confidence with the main concepts of precision Graph Neural Networks and its application to Genomics. For the second quarter, we will split into smaller groups which will independently build on top of the concepts developed in the first quarter. At the end of Quarter 1, we expect having a paper to be published in Re:Science. Students will work on publicly available datasets and will be doing all the analysis on their own. GitHub will be used as the primary channel to report results and progress. Students will be expected to know basic concepts of neural networks, such as how back-propagation works, how to change meta-parameters such as learning rate, etc. Students are not required to understand in details how Graph Neural Networks work, as will save time to study this topic in Quarter 1. At the end of Quarter 1, we expect having a paper to be published in Re:Science.
- Suggested Prerequisites: Background in Genomics (basic), background in Neural Networks (training small feedforward networks/MLP)
- Summer Tasks: https://arxiv.org/pdf/2312.02783 https://www.cell.com/iscience/pdf/S2589-0042(23)00308-5.pdf Familiarity with PyTorch, Tensorflow, Pandas, Networkx, Deep Graph Library
🤝 Fairness and Causal Inference
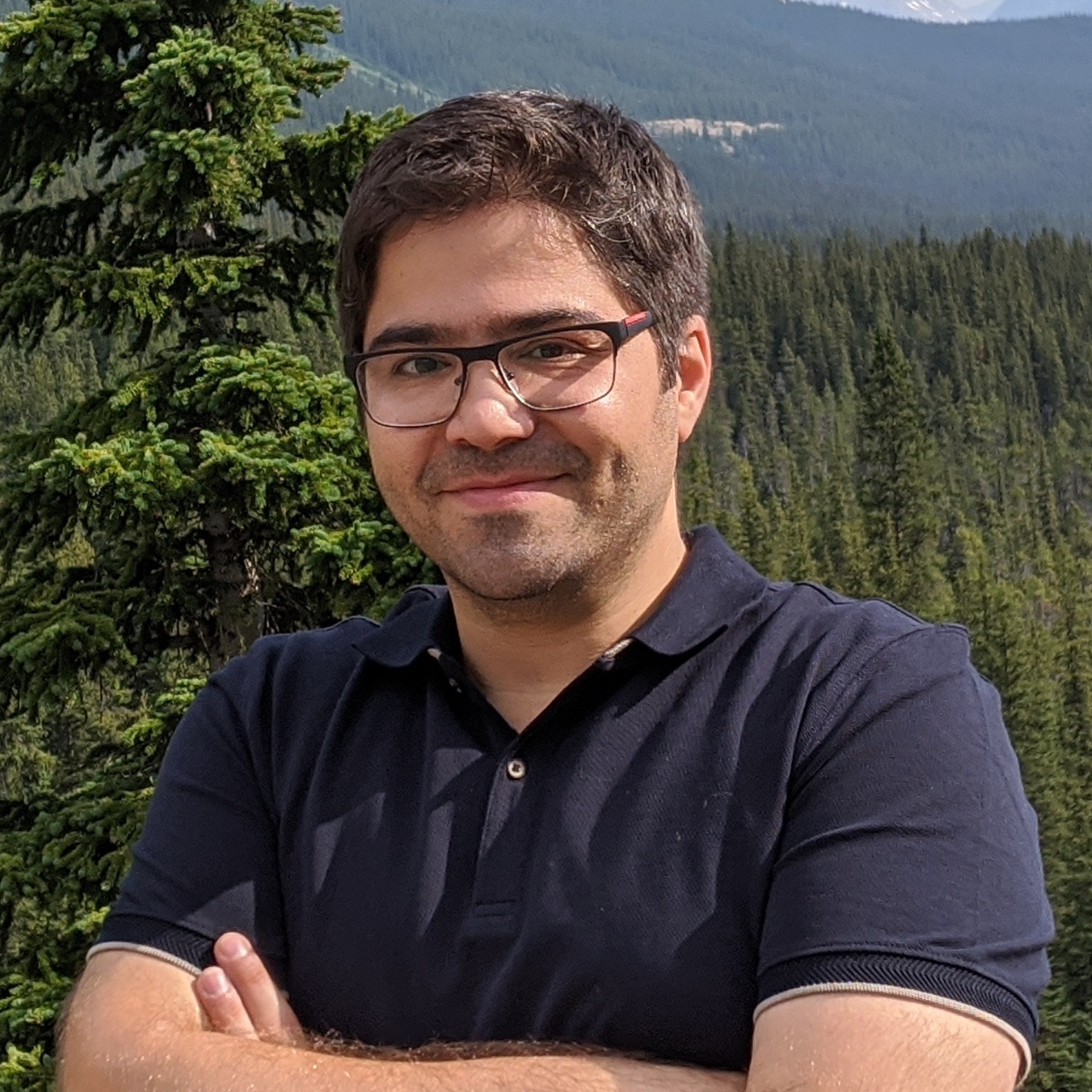
Data-Centric AI: Data Quality Management for Improved Fairness, Robustness, and Accuracy in Predictive Modeling
Babak Salimi • bsalimi@ucsd.edu
A07 6 seats Friday 3-4PM, Hybrid
In the rapidly evolving field of artificial intelligence, the integrity and quality of data play pivotal roles in determining the effectiveness and ethical impact of AI systems. This domain focuses on the critical need for Data Quality Management in AI, specifically aimed at enhancing fairness, robustness, and accuracy in algorithmic decisions. As AI technologies become increasingly integrated into various sectors—ranging from healthcare to finance—the demand for models that not only perform consistently across diverse conditions but also maintain equitable outcomes is paramount. Students exploring this domain will delve into methodologies and strategies to assess, refine, and augment data quality. By investigating how data anomalies, biases, and inconsistencies affect model performance, students will be equipped to propose innovative solutions that ensure AI systems are both technically sound and socially responsible. The ultimate goal is to prepare students to develop AI applications that are capable of standing up to real-world challenges and ethical scrutiny, making them valuable across different industries and societal contexts.
Read more
- About: My research interests focus on advancing the field of trustworthy data analysis by fostering responsible data management practices. I am deeply passionate about data management, as I believe that having reliable, accessible, and well-organized data is crucial for establishing trust in data-driven decision-making. My research aims to create methods that promote transparency, fairness, reliability, and robustness in algorithmic decision-making processes. By adopting a data management-centric approach, I strive to develop tools and techniques that empower human decision-makers to interpret data more accurately and confidently. In my research group, we are committed to creating tools that support decision-makers from diverse backgrounds in understanding data and making more informed choices. Our goal is to enable better decision-making by bridging the gap between complex data and human understanding, ultimately fostering trust in data analysis.
- Mentoring Style: As your mentor, I'll guide you through the basics and help you understand the relevant material, creating a solid foundation for your projects. While I’ll provide close support and clear explanations of complex concepts, you'll have the opportunity to take the lead on your project, making key decisions and steering its direction.
- Suggested Prerequisites: Machine learning, scalable analytics, probability, inference
- Summer Tasks: https://docs.google.com/document/d/1YaPLOHUX2X84MzaWfT4y0P4Jg3-ksCMa5qB3RuYl1io/edit
- Previous Project
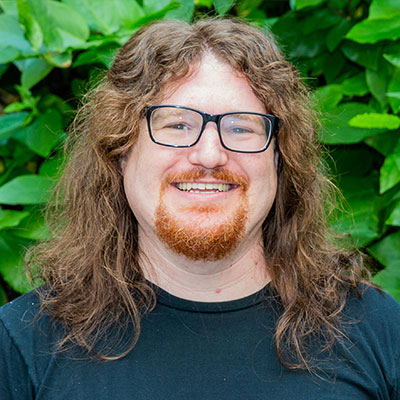
Community-Centered Discrimination Audits of LLMs: Bias Rapid Action Teams
Stuart Geiger • sgeiger@ucsd.edu
A08 4 seats Wednesday 10-11AM, In-Person
This capstone will work with community members to audit pretrained Large Language Models for discrimination and bias, using perturbation-based and controlled-experimental methods. These systematically vary a template prompt along a potential type of discrimination, then observe differences in outputs. For example, if you ask ChatGPT (or TritonGPT) to act as a college admissions reviewer, does an application's score change if it references the Mens vs Womens basketball team? Or being on the lacrosse versus basketball team? Or being from different hometowns? These methods are relatively simple from a data science and statistics perspective. The hard part is knowing what kinds of discrimination are of most concern to the people who will be impacted by model outputs, then creating real-world template prompts that test for those concerns. This capstone will be centered around **talking and listening to real people** about their concerns with LLMs in real-world contexts, then using our data science expertise in a more consulting-style mode. If a team chooses university admissions, they might work with students, high school councilors, professors, and/or admissions staff. All students must take and pass the 3-hour UCSD/CITI IRB Human Subject Protection Training online course (Social and Behavioral Basic) by week 3 of Fall.
Read more
- About: I’m an social scientist with a background in the humanities, especially history and philosophy of science and technology, but I have enough expertise in computer science and data science to make trouble. I believe that data science systems should be fair, transparent, and accountable to the public, but that most are currently not. A lot of my research is in community-centered content moderation NLP systems for user-generated content, especially Wikipedia, where I formerly worked on their ML models and systems.
- Mentoring Style: I will be the point of contact and there every week, but may bring in collaborators and my grad student advisees. I intentionally do not run a "lab", but I do have a "constellation of collaboration." Students can choose their own particular context in which LLMs are deployed and which kinds of community members / impacted people they want to consult.
- Suggested Prerequisites: Base data science prereqs are sufficient (we won't dive deep into foundations of LLMs or causal inference). We will be talking and listening to people (especially strangers) and serving as their ad-hoc consultants. Skills in consulting, volunteering, tabling for student orgs, etc. are not required, but will be useful -- and you will build those skills if you do not have them.
- Summer Tasks: Read:
- A recent example of a perturbation-based audit study: https://arxiv.org/pdf/2402.14875
- Our recent paper on why audits must be community-centered and how this kind of auditing relates to democratic governance: https://escholarship.org/content/qt6r820956/qt6r820956.pdf
- For more readings, see: https://auditlab.stuartgeiger.com
- Take UCSD/CITI IRB Human Subject Protection Training online course (Social and Behavioral Basic), must complete by week 3 of Fall, but good to do it earlier. Register at citiprogram.org and see this video for how to register: https://www.youtube.com/watch?v=hOAgfK93QXg
- Get familiar with OpenAI API and ollama (https://ollama.com/) for self-hosted open-source LLMs (which uses OpenAI API schema)
- Previous Project
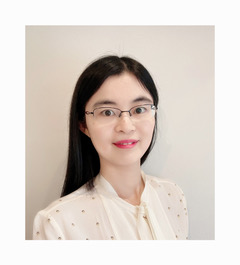
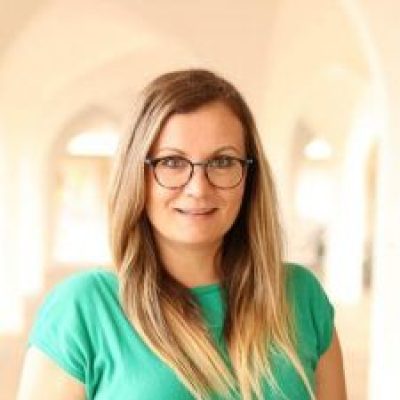
Learning and Using Causal Knowledge for Advancing AI and Scientific Discovery
Biwei Huang and Jelena Bradic • bih007@ucsd.edu
and jbradic@ucsd.edu
A09 10 seats Friday 10:30-11:30AM, Zoom
Causal information is essential in many tasks in empirical sciences and engineering. For example, in medical science, to find out an effective, reliable treatment for Alzheimer’s disease, it is crucial to find the underlying genetic factors that are responsible for the disease and figure out how they jointly influence the disease. In AI, to achieve general-purpose AI with the capacity of reasoning, acting, and generalizing to novel domains or tasks, one challenge is to move beyond domain-specific pattern recognition towards the discovery and use of underlying causal relationships that produce stable and interpretable patterns across general scenarios of interest. To this end, two questions naturally arise: how can one acquire causal knowledge and, furthermore, how should we use it? Accordingly, we will focus on these two questions and study tools on (1) automated causal discovery and inference from non-experimental data in complex environments, (2) advancing machine learning from the causal perspective, and (3) using or adapting causal discovery and inference approaches to solve scientific problems.
Read more
- About: Biwei Huang is an Assistant Professor at HDSI. Previously, I received my Ph.D degree from CMU. My research interests include causal discovery and inference, causality-related machine learning, and computational science.
Jelena Bradic is a Full Professor at the UC San Diego, where she holds a joint appointment in the Department of Mathematical Sciences and Halicioglu Data Science Institute. Prof. Bradic's interests are in causal inference, machine learning, robust statistics as well as missing data problems. Her application areas include observational and interventional data, treatment effects, as well as public health and policy learning. She strives to understand and develop new robust learning methods and algorithms with provable guarantees of stability, robustness to data corruption and data generating mechanism. - Mentoring Style: We will suggest project ideas and guide students through the steps required to complete them. We will adapt our level of involvement based on the individual needs of each student.
- Suggested Prerequisites: None
- Summer Tasks: 1. Read the first two chapters of the following book: Causal Inference in Statistics - A Primer. By Judea Pearl, Madelyn Glymour, Nicholas P. Jewell
2. Read the 5th chapter of the following book: Spirtes, P., Glymour, C. N. & Scheines, R (2000). Causation, prediction, and search. MIT press.
3. Read the first three chapters of the following book: James Robins and Miguel A. Hernan. 2010. Causal Inference: What If.
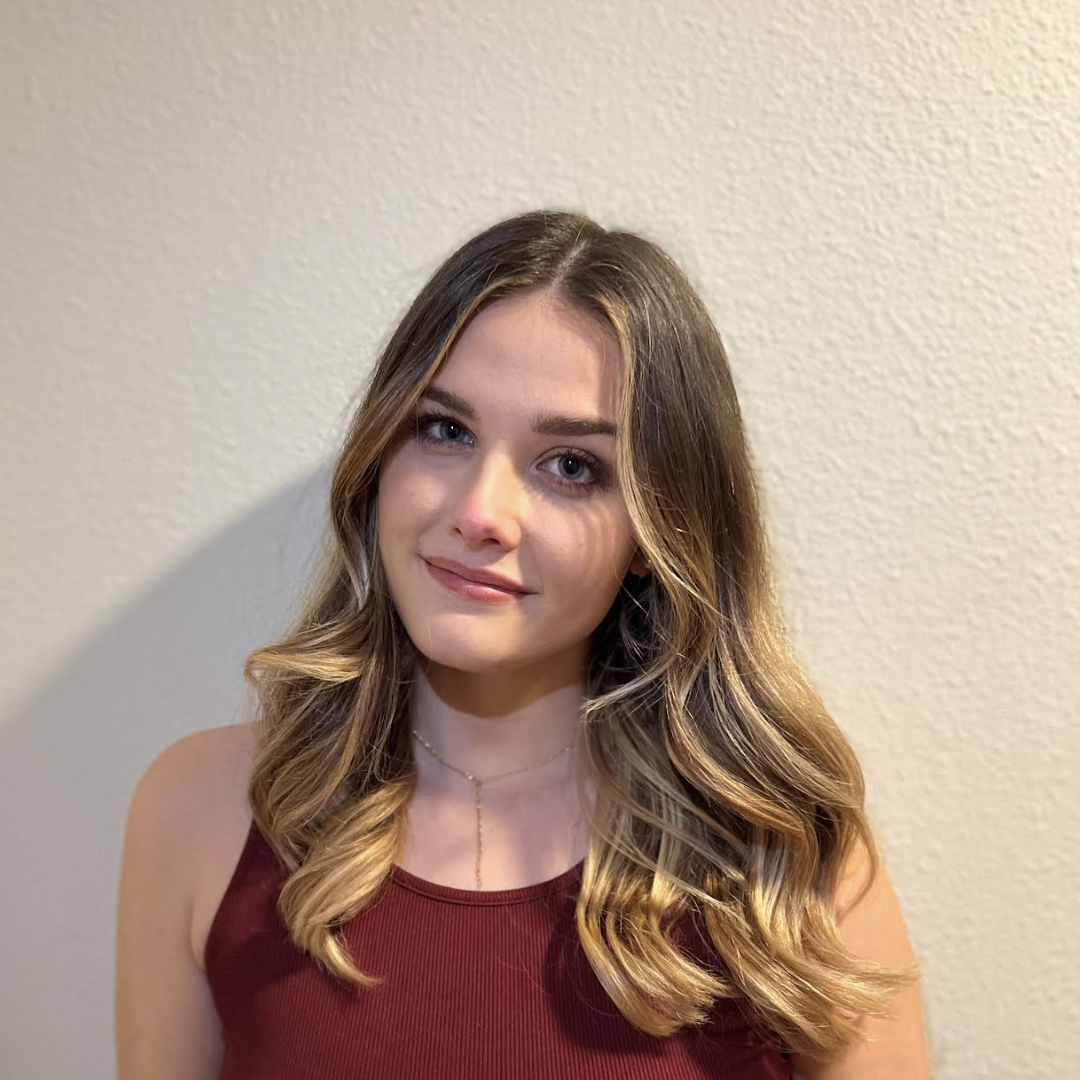
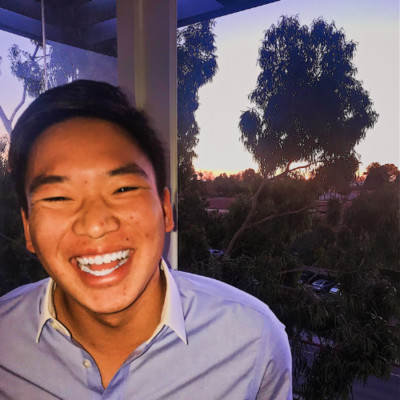
Ethical Considerations in Using Artificial Intelligence
Emily Ramond and Greg Thein • With questions for industry partners, email Suraj
A10 10 seats Tuesday 2-3PM, Zoom
Industry Partner: Deloitte
There is a growing societal concern over the potential and real negative effects of AI, particularly in terms of fairness and explainability. This concern is considered in this course where students will study high-profile cases of algorithmic discrimination, explore different definitions and metrics of AI fairness, and understand their practical implications. The challenge lies in translating these complex concepts into real-world applications, training students to independently analyze AI fairness and explainability, and emphasizing the societal impact of these issues. The course aims to equip students with skills to assess algorithmic fairness, understand data limitations, and apply bias mitigation techniques in AI models. Students will explore the ethical dimensions of artificial intelligence (AI), with a specific focus on fairness assessments and bias mitigation. This course integrates practical workshops, case studies, include IBM AI Fairness 360 Model Overview and the evaluation of model bias using Medical Expenditure data. Through lectures, workshops, readings, and hands-on projects, students will gain an understanding of how to assess algorithmic fairness, measure fairness metrics, and identify the limitations of data in capturing fairness. They will also learn techniques for mitigating bias in AI models through pre-, in-, and post-processing. The course will emphasize real-world applications and the impact of ethical AI considerations on different stakeholders. Students will engage in replication projects and independent analyses to develop their skills in fairness assessments and bias mitigation.
Read more
- About: Greg completed his undergraduate studies at HDSI in 2021, where he was an active member of the ERC community. His capstone project centered around Alzheimer's gene analysis. After graduating, Greg joined Deloitte as a Business Technology Analyst, where he engages in diverse tasks encompassing data management, analytics, and dashboarding for various clients. In his free time, Greg loves to travel, explore new restaurants and bakeries, and play sports/working out (tennis, swimming, and snowboarding). As the AI space grows and evolves, Greg is passionate about ensuring products and models are built with ethical considerations in mind, allowing for greater data driven and technological integrations within society. Emily completed her undergraduate studies at HDSI in 2022, where she was an active member of Marshall College. Her capstone project centered around causal inference. Post-graduation, Emily joined Deloitte as a Business Technology Analyst. In this role, she engaged in diverse tasks encompassing data analytics, machine learning, and engineering for a wide array of clients. Beyond academic and professional pursuits, Emily loves crocheting, travel, snowboarding, and fostering cats. Drawing inspiration from her coursework at Marshall College, Emily is passionate about ethical artificial intelligence. Her commitment extends to prioritizing fairness, transparency, and accountability. She is driven by her interest in leveraging the power of data science for the betterment of the world.
- Mentoring Style: Q1 will be held in a typical classroom format. Everyone shows up to class and participates. Each student will need to present once on a reading (5 min presentation). We will have frequent class discussions and the replication project will be two groups of 5. We may have guest lecturers. Q2 is much more independent. We will hold one hour meets for EACH group (up to 4 people per group) once a week to check in on progress. Occasionally, we will have additional office hours as needed. You will be completing a project start to finish - from picking domain and gathering data to creating a report and website to present. We cannot guarantee we have knowledge in the domain - so be prepared to do research. We will be on Discord and present throughout the entire process.
- Suggested Prerequisites: None
- Summer Tasks: Read Deloitte's Trustworthy AI Website: https://www2.deloitte.com/us/en/pages/deloitte-analytics/solutions/ethics-of-ai-framework.html Familiarize yourself with IBM's AIF360 Demo: https://aif360.res.ibm.com/ Proficient in python (review DSC80 work - missingness, EDA, hyperparameter tuning, visualization best practices, etc)
- Previous Project
đź§ Theoretical Foundations
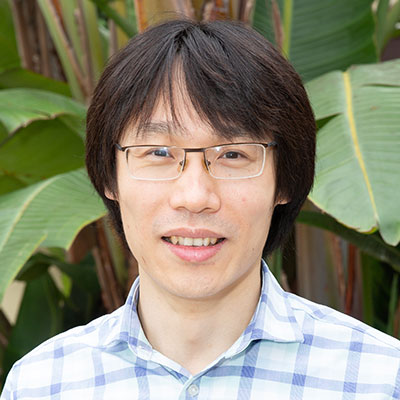
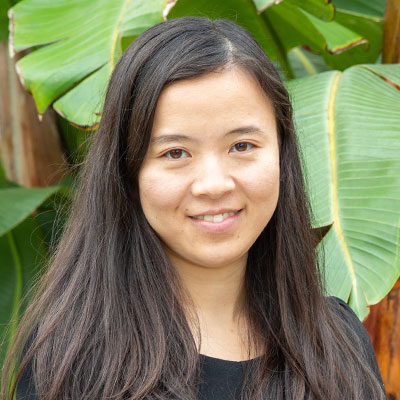
Probabilistic Deep Sequence Models for Bayesian Optimization
Yian Ma and Rose Yu • yianma@ucsd.edu
and roseyu@ucsd.edu
A11 6 seats Tuesday or Thursday Afternoon, Zoom
Decision-making under uncertainty requires models that can generate not only point estimates but also confidence intervals. We investigate deep sequence models for Bayesian optimization in spatiotemporal domain, with the goal to reduce sample complexity, provide risk assessment, and guide policy making.
Read more
- About: Rose Yu is an assistant professor at UC San Diego department of Computer Science and Engineering. She is a primary faculty with the AI Group and is affiliated with Halıcıoğlu Data Science Institute.
Her research interests lie primarily in machine learning, especially for large-scale spatiotemporal data. She is particularly excited about AI for scientific discovery. She has won ECASE Award, NSF CAREER Award, Hellman Fellowship, Faculty Awards from JP Morgan, Meta, Google, Amazon, and Adobe, several Best Paper Awards, and Best Dissertation Award at USC.
Yian Ma is an assistant professor at the Halıcıoğlu Data Science Institute and an affliated faculty member at the Computer Science and Engineering Department of University of California San Diego. Prior to UCSD, he spent a year as a visiting faculty at Google Research. His current research primarily revolves around scalable inference methods for credible machine learning. This involves designing Bayesian inference methods to quantify uncertainty in the predictions of complex models; understanding computational and statistical guarantees of inference algorithms; and leveraging these scalable algorithms to learn from time series data and perform sequential decision making tasks. - Mentoring Style: Postdocs and PhD students will help mentor
- Suggested Prerequisites: CSE 151B/251B
- Summer Tasks: Please read the following two papers and get familiar with the open source code in the experiment sections:
https://arxiv.org/abs/2305.04392
https://arxiv.org/abs/2402.18846 - Previous Project
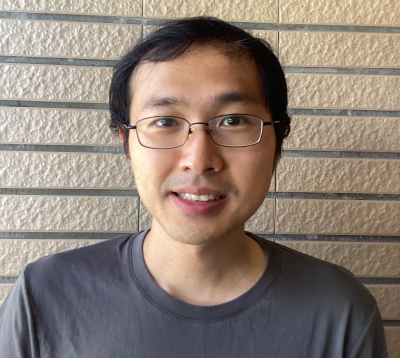
Tackling Distribution Shifts via Test-Time Adaptation and Optimization
Jun-Kun Wang • jkw005@ucsd.edu
A12 4 seats Friday 1-2PM, In-Person
Tackling the problems of machine learning under distribution shifts has drawn great interest due to the emerging concern regarding the reliability of machine learning techniques when applied to real-world systems, where distribution shifts between training and testing data are often unavoidable. For example, in medical applications, machine learning model were typically trained on data that were collected from some specific institutions, while the model is adopted by institutions outside the training set, and hence distribution shifts naturally occur. The challenges of distribution shifts also arise in many other fields, e.g., robotics, ML for education, ML for agriculture, or ML for wildlife monitoring, to name just a few.
Test-time adaptation is a task for tackling distribution shifts. It refers to adapting a model from a source-domain to a new domain at test time, where only unlabeled samples from the new domain are accessible. Its applications include predictions on sensor data, climate data, medical images, in which distribution shifts of data could occur at test time and annotating the labels could be costly. A common approach in test-time adaptation is constructing pseudo-labels for those unlabeled samples and using optimization methods like gradient descent to minimize a certain loss function with the pseudo-labels to update the model. In this capstone project, we will leverage optimization techniques to speed up adaptation to the new domain. Students will have substantial hands-on (PyTorch) experiences, from reproducing existing algorithms to designing and implementing their own methods.
Read more
- About: I am an assistant professor at HDSI and ECE. My research is centered around optimization and its connections with statistics and machine learning.
- Mentoring Style: Students will be expected to use Python/PyTorch to implement their algorithms (and should be able to code).
- Suggested Prerequisites: Optimization will be fundamental to this capstone project. It is *highly recommended* to take an undergraduate-level optimization course in the Fall quarter, e.g., ECE 174 Intro/Linear&Nonlinear Optimization or MATH 173A Optimization/Data Science I
- Summer Tasks: Complete reading the following three relevant papers:
Continual Test-Time Domain Adaptation Qin Wang, Olga Fink, Luc Van Gool, Dengxin Dai https://arxiv.org/abs/2203.13591 CVPR 2022
On Pitfalls of Test-Time Adaptation Hao Zhao, Yuejiang Liu, Alexandre Alahi, Tao Lin ICML 2023 https://proceedings.mlr.press/v202/zhao23d/zhao23d.pdf
Test Time Adaptation via Conjugate Pseudo-labels Sachin Goyal, Mingjie Sun, Aditi Raghunathan, J. Zico Kolter https://arxiv.org/abs/2207.09640 NeurIPS 2022
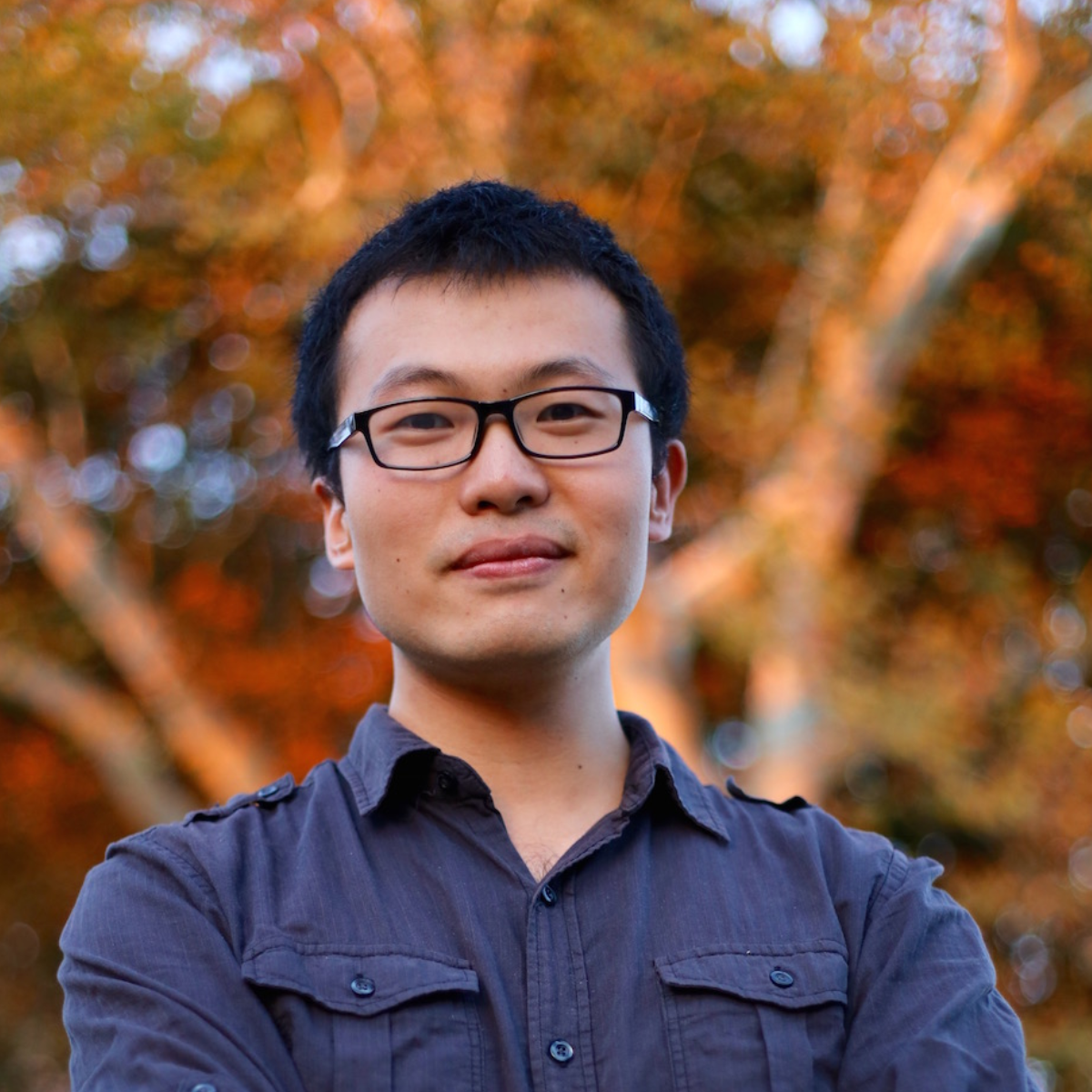
Design Machine Learning Methods that are Scalable, Effective, and Come with Provable Guarantees
Yu-Xiang Wang • yuw272@ucsd.edu
A13 6 seats Friday Morning, In-Person
My research interest is broad, but I prefer students explore either of the following two domains.
Domain 1: Differentially private data science. Many data science problems involve handling sensitive data of individual subjects. Even if the personal identifiable informations (PIIs) are removed, individuals can still be re-identified using the output ML models, its predictions and even merely summary statistics --- especially when combined with side information. While the theory of private learning is well-developed, other important aspects of data science, e.g., data-preprocessing, missing-data imputation and model selection are less explored. The research problem may involve developing new differentially private methods for these tasks and conducting end-to-end data analysis tasks on specific applied problems, e.g., predicting disease using electronic patient records or controlling blood glucose level for diabetes patients.
Domain 2: Evaluation and Attack on LLM watermarks. Watermarking is a promising approach towards addressing the LLM abuse. It injects subtle statistical signals in the LLM generated text that makes it detectable when a secret key is given. However, the statistical signal can be weakened or even removed if the generated text is edited, paraphrased or otherwise post-processed. The general scope of this project is to come up with practical attacks on existing watermarking schemes so as to evaluate how useful they are in practice.
Read more
- About: Associate Professor at HDSI, affiliated with CSE. Research focuses on statistical machine learning, differential privacy, reinforcement learning, optimization and all kinds of applications. Recently, I am most excited about theory of deep learning and watermarking large language models. An ideal undergraduate project would be one that investigates a particular applied problem with a concrete dataset available using techniques develops from my lab.
- Mentoring Style: Each project will have a PhD student mentor assigned who will offer additional office hours each week. Students will be able to reach the faculty and graduate student mentors on Slack too. To make the weekly session effective, students are expected to devote time to complete independent work each week.
- Suggested Prerequisites: Knowing statistics and optimization theory will help with reading technical papers from my group. For projects that involves coding and experimentation, it is important for the student to have taken a first course in data structures and algorithms.
- Summer Tasks: Working on the above prerequisites. Reading papers. Watching my recorded lectures.
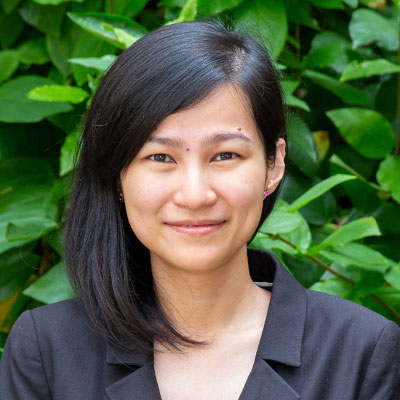
Robust and Interpretable Neural Network Models for Computer Vision and Natural Language Processing
Lily Weng • lweng@ucsd.edu
A14 6 seats Monday 4-5PM, Zoom
The goal of this project is for students interested in robustness and interpretability for deep neural network models. Students will develop methods to improve robustness and interpretability of deep learning tasks such as computer vision and natural language processing.
Read more
- About: Lily Weng is an assistant professor in Halıcıoğlu Data Science Institute with affiliation to Computer Science and Engineering Department at UC San Diego. Her research vision is to make the next generation AI systems and deep learning algorithms more robust, reliable, explainable, trustworthy and safer. More details please see lilywenglab.github.io
- Mentoring Style: This project will be purely research-oriented and heavier than the usual course project. Students are expected to lead their capstone project under Prof. Weng's guidance. Students who have successfully completed DSC 140B, and familiar with deep learning algorithms in computer vision or natural language processing, and deep learning libraries (e.g. pytorch) and neural networks are more likely to succeed in this project.
- Suggested Prerequisites: DSC 140A, DSC 140B, DSC 190 Trustworthy Machine Learning, CSE 151A, CSE 151B, CSE 150A, CSE 150B, CSE 152A, CSE 152B, CSE 156
- Summer Tasks: If you are able to understand below papers and setup the code repo provided in below, then you are likely to succeed in this capstone project. If you have problems setting up the repo or/and understand the technical details in below papers, then this capstone session is very likely not a good fit for you.
Please don't be discouraged, it only means that you require more background e.g. you are encouraged to take Prof. Weng's DSC 190 Trustworthy machine learning, also DSC 140A, DSC 140B, CSE 150-152, CSE 156.
Required Reading before 1st class in Fall 24: 1. https://arxiv.org/pdf/2204.10965 2. https://arxiv.org/pdf/2304.06129 3. https://arxiv.org/pdf/2304.13346.pdf, 4. https://arxiv.org/abs/2403.13771, project website: https://lilywenglab.github.io/Describe-and-Dissect/ 5. https://arxiv.org/pdf/2310.06200, project website: https://lilywenglab.github.io/Efficient-LLM-automated-interpretability/
Required reproduced results before 1st class in Fall 24: 1. https://github.com/Trustworthy-ML-Lab/CLIP-dissect 2. https://github.com/Trustworthy-ML-Lab/Label-free-CBM 3. https://github.com/Trustworthy-ML-Lab/Efficient-LLM-automated-interpretability - Previous Project
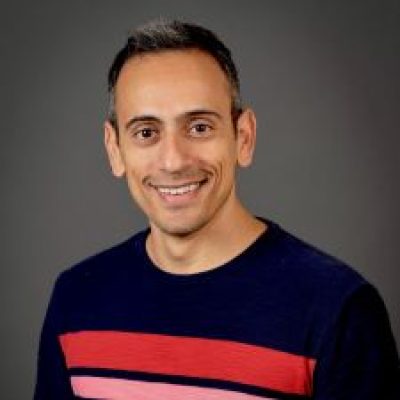
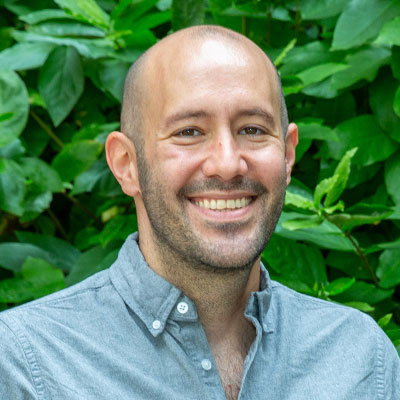
Neural Network Compression with Error Guarantees
Rayan Saab and Alex Cloninger • rsaab@ucsd.edu
and acloninger@ucsd.edu
A15 8 seats Monday 2-3PM, In-Person
While deep learning systems can solve a large number of problems, their ever-growing computational demands pose a challenge for deployment on resource-constrained platforms such as cell phones and small chips. To facilitate moving ML systems to edge computing, it is imperative to reduce their number of active weights and to reduce the computational load associated with them. A number of approaches are plausible. Among these, sparsification seeks to reduce the number of active weights by setting as many of them to zero as possible, while quantization replaces, for example, 32-bit floating point weights with weights that require many fewer bits to store. Despite numerous proposed methods for weight sparsification and quantization, only a handful offer theoretical guarantees that predictions using quantized weights will closely approximate those of the original large network.
The domain of this project will cover methods of sparsification and quantization that guarantee the resulting error will remain small, alongside examining their applications across diverse deep learning architectures, including those for computer vision and large language models. It will involve a balanced mixture of theory and practice. Students who choose this project will delve into the mathematical and computational principles behind quantization, utilizing concepts from signal processing, statistical learning, and linear algebra. They will also engage in hands-on coding and experimentation on algorithms for compressing deep learning models, testing them on various data sets and signal models.
Read more
- About: Alex Cloninger is an Associate Professor in Mathematics and the Halicioglu Data Science Institute. He works on computational models for learning similarities between data, and using these similarity measures to solve various scientific problems. Find out more about Dr. Cloninger's research: https://ccom.ucsd.edu/~acloninger/index.html
Rayan Saab is a Professor in the Mathematics Department and at the Halicioglu Data Science Institute. He works on developing computational methods and theory for solving problems related to collecting, processing, and analyzing data. He came to this work first through an undergrad degree in electrical engineering and finding himself always interested in both making things work and understanding why they do. Find out more about Dr. Saab's research: http://www.math.ucsd.edu/~rsaab/ - Mentoring Style: We both are relatively hands-on in the sense that we make ourselves available for problem-solving and discussions. That said, students have to be self-motivated, and motivated to do the readings and the work.
- Suggested Prerequisites: Being comfortable with probability and linear algebra (or willingness to catch up quickly) would be very helpful, as would be a basic familiarity with neural networks.
- Summer Tasks: Here are some relevant readings. Students need not go into the mathematical details of the papers as we can go through them together, but these papers give an idea of the domain. The more familiar you are with the topic, the more we can do!
https://arxiv.org/abs/2201.11113
https://proceedings.mlr.press/v119/elthakeb20a.html
https://arxiv.org/abs/2210.17323
To be able to obtain really nice experimental results, you'll need to pick up PyTorch and work with the ImageNet dataset. - Previous Project
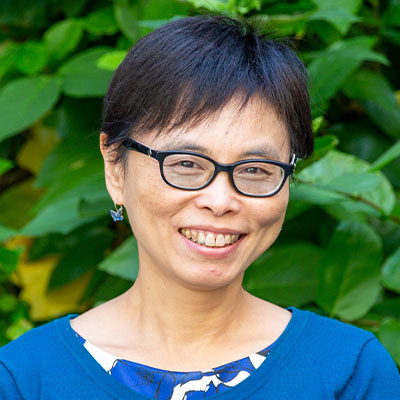
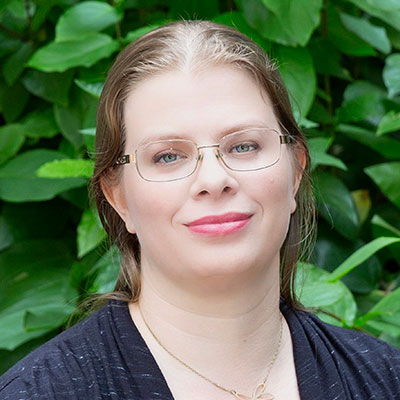
How Effective are Transformer Based Graph Learning Models?
Yusu Wang and Gal Mishne • yusuwang@ucsd.edu
and gmishne@ucsd.edu
A16 10 seats Wednesday 9-10AM, In-Person
Graph data are ubiquitous across a broad range of applications in science and engineering. In recent years, there has been a tremendous amount of development in efficient neural network models for learning and optimization on graphs. Two families of popular models are: Message passing graph neural networks, and Graph transformer-based models. In particular, with the success of transformer architectures in many other types of data, especially in large language models, it is natural to ask whether graph-transformers can achieve a similar success. On the other hand, originally transformers are not defined for graph data, and in order to use them to handle graph (or point-cloud data), one has to inject graph topology into the model in some way. The ultimate goal of this project is to explore the relative pros and cons of using different graph transformer models for graph learning tasks. In Quarter 1, the students will get familiar with several baseline graph transformer models and understand the underlying principles. In Quarter 2, they will work on different (potentially novel) ways to inject graph information to the transformer and compare the performance, and potentially apply to novel data sets and tasks.
Read more
- About: Yusu Wang is a professor in HDSI. She is primarily interested in geometric and topological data analysis, especially graph learning, geometric deep learning, and so on. In general, she would like to develop efficient and effective learning models for complex data, and graphs (as well as point clouds data) constitute one particular type of data that she is interested in.
Gal Mishne is an assistant professor in HDSI. Her research is on geometric data analysis and focuses on modeling data as lying on a graph or being sampled from a (nonlinear) manifold. Her research group develops methods that take this geometry into account in order to process, analyze, and visualize high-dimensional data. She primarily collaborates with neuroscientists and other biomedical researchers, to apply models and methods to real-world data. - Mentoring Style: We expect students to be self motivated to do the reading and coding tasks in Q1 and to take ownership of their projects in Q2, with our support. Students are expected to treat the project seriously and devote sufficient time to making weekly progress toward their goals. We are always happy to discuss and help problem-solve.
- Suggested Prerequisites: Students should already have experience with neural network models (e.g., CNNs, RNNs, or best but not required, GNNs). Solid knowledge of linear algebra and graph theory is preferred.
- Summer Tasks: Please check out pytorch geometric (https://pytorch-geometric.readthedocs.io/en/latest/) on graph learning models, read about simple models such as GCN and GAT.
- Previous Project
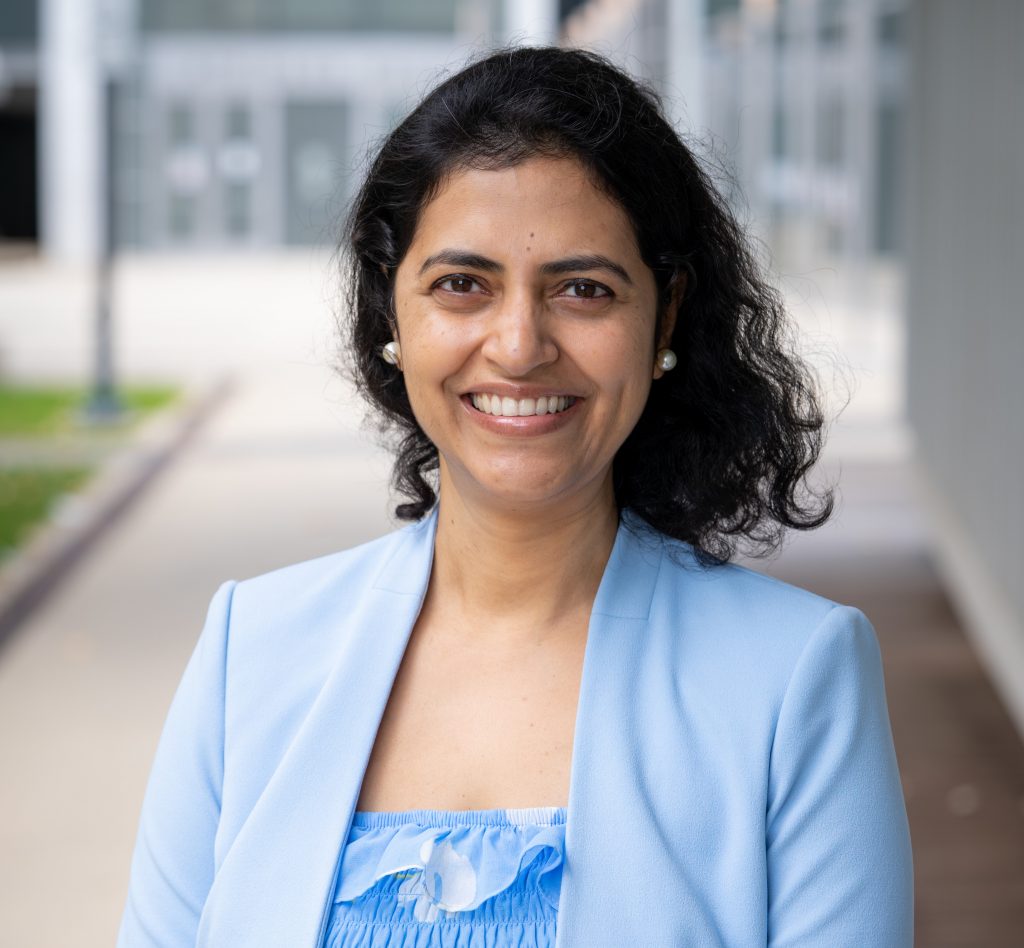
Graph Algorithms
Barna Saha • bsaha@ucsd.edu
A17 4 seats Tuesday Morning, Zoom
Students will learn some state of the art algorithms to deal with massive graphs that occur in the context of social networks.
Read more
- About: Director of the National NSF TRIPODS Institute for Emerging CORE Methods in Data Science (EnCORE)
The Harry E. Gruber Endowed Chair Professor of Computer Science and Information Technologies, University of California San Diego
Department of Computer Science & Engineering, and Halıcıoğlu Data Science Institute
Previously, I was a tenured Associate Professor at the University of California Berkeley, and even before that on the faculty of Computer Science at UMass Amherst, and as a Senior Research Scientist at the AT&T Shannon Research Laboratory. I am also an Affiliate faculty of the Simons Institute for the Theory of Computing at UC Berkeley. - Mentoring Style:
- Suggested Prerequisites:
- Summer Tasks:
- Previous Project
🗣️ Language Models
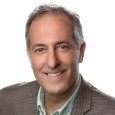
GenAI for Good
Ali Arsanjani • arsanjani@google.com
B01 8 seats Thursday 3:30-5PM, In-Person
Generative AI for Good refers to the application of generative artificial intelligence (AI) techniques to address societal challenges and promote positive outcomes. In the context of misinformation and disinformation detection and mitigation, it involves leveraging generative AI models to combat the spread of false or misleading information and reduce socio-political polarization. Generative AI models, such as language models and deep learning algorithms, have shown remarkable capabilities in generating text and content that closely resembles human-produced content. These models can be trained to understand and analyze large amounts of data, including news articles, social media posts, and online discussions, to detect patterns and identify potential misinformation or disinformation. By employing generative AI techniques, it becomes possible to develop sophisticated algorithms and systems that can automatically identify false or misleading information, distinguish it from accurate information, and mitigate its impact on public opinion and discourse. These systems can analyze the content, context, and sources of information, looking for inconsistencies, logical fallacies, and biases that are indicative of misinformation. Generative AI can also play a crucial role in reducing socio-political polarization by promoting more balanced and factual narratives. By identifying and flagging content that contributes to polarization, algorithms can provide users with alternative viewpoints, fact-checking information, or context that helps to counterbalance the biases inherent in some narratives. This can encourage critical thinking, promote a more informed public, and foster constructive dialogue across diverse perspectives. However, it is important to note that generative AI techniques are not without challenges. Ensuring the accuracy and fairness of these models, avoiding biases, and balancing freedom of expression with the need to combat misinformation are critical considerations. Ethical guidelines and rigorous validation processes should be put in place to address these concerns and ensure the responsible and effective deployment of generative AI for good in the context of misinformation and disinformation detection and mitigation. alternusvera.com
Read more
- About: www.linkedin.com/in/ali-arsanjani
- Mentoring Style: As a formal course, and in teams
- Suggested Prerequisites: NLP
- Summer Tasks: Learn NLP, especially with large language models from Google using Google AI Studio.
- Previous Project
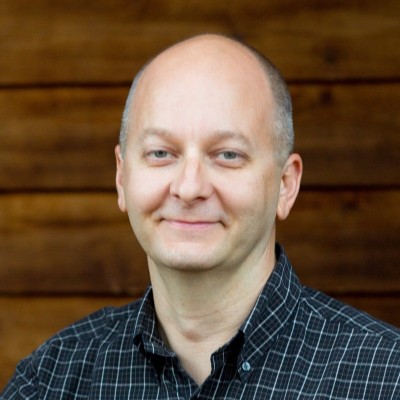
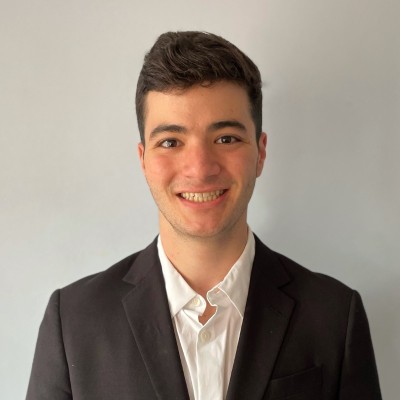
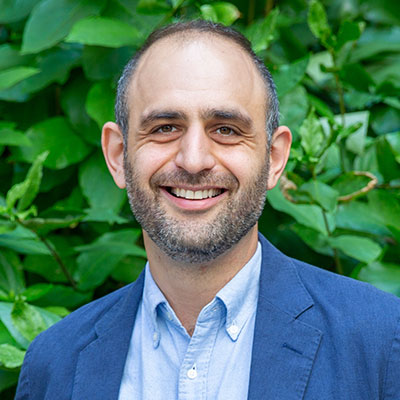
NLP Credit Score Development
Brian Duke, Kyle Nero, and Berk Ustun • With questions for industry partners, email Suraj
and berk@ucsd.edu
B02 10 seats Friday 10-11AM, Hybrid
Industry Partner: Prism Data
One of the most widely used and little understood parts of the Financial Services industry is the credit score. In this course, students will work with transactional bank data to build statistical models for the purpose of assessing creditworthiness in the financial services industry. The course will take students through the life of a model development project, from data exploration, through model training and evaluation. Students will have the opportunity to work with both structured and unstructured data as they learn about the process and attributes that go into credit scores. Additionally, students will learn about the importance of model explainability and fairness.
Read more
- About: Brian Duke has been a data scientist for 23 years in the Financial Services industry. He has worked at Capital One, FICO, SAS Institute, Accenture, Experian, Petal Card and currently is the Head of Data Science at Prism Data. A common theme in his work has been translating transactional data into useful scores and analytical insights for use in risk decisioning. Brian received his BA and MS from the University of California, San Diego and continues to reside in the San Diego area today. He holds 4 patents and has 12 pending in the United States.
Berk Ustun's research lies at the intersection of machine learning, optimization, and human-centered design. His group develops methods for responsible machine learning in medicine, consumer finance, and the physical sciences. They focus on topics like algorithmic fairness, interpretability, and personalization. Previously, he held research positions at Google and at the Harvard Center for Research on Computation and Society. Berk received a PhD in Computer Science from MIT, and Bachelors degrees in Operations Research and Economics from UC Berkeley. - Mentoring Style: Our group will consist of group projects completed in groups of 3-4. The goal of the course is to eventually build a credit score but we will start by building a transaction categorization model using NLP techniques. Each week we will talk about techniques that can be applied to the next step in the project. We will begin by reviewing homework from the previous week and discussing ideas. Then introduce the next step and talk about what can be done to solve the next step in the problem. The goal is to introduce students to the model development process in most financial services companies.
- Suggested Prerequisites: DSC140A, DSC140B
- Summer Tasks: https://www.capitalone.com/learn-grow/money-management/when-did-credit-scores-start/
https://www.capitalone.com/learn-grow/money-management/fair-credit-reporting-act/
https://www.capitalone.com/learn-grow/money-management/equal-credit-opportunity-act/
https://www.nerdwallet.com/article/finance/credit-score-ranges-and-how-to-improve
https://developers.google.com/machine-learning/crash-course/classification/roc-and-auc#:~:text=An%20ROC%20curve%20(receiver%20operating,False%20Positive%20Rate
- Previous Project
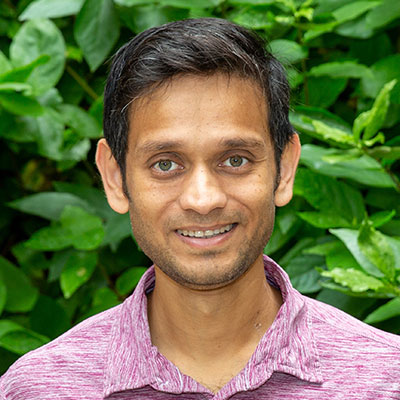
Quantization and Sparsification in LLMs
Arya Mazumdar • amazumdar@ucsd.edu
B03 6 seats Tuesday 11AM-12PM, In-Person
How do you quantize the trained weights of a neural network to get fast inference in a large scale machine learning model? Should you just use the same quantizer for all layers? How do you develop the theory for quantization in LLMs? How do you quantize the trained weights of a neural network to get fast inference in a large scale machine learning model? Should you just use the same quantizer for all layers? How do you develop the theory for quantization in LLMs?
Read more
- About: Arya Mazumdar is an Associate Professor of Data Science in UC San Diego. He is the Deputy Director and the Associate Director for Research in the NSF AI Institute TILOS, and also the UCSD Site-Lead of NSF TRIPODS Institute EnCORE. Arya obtained his Ph.D. degree from University of Maryland, College Park specializing in information theory. Subsequently Arya was a postdoctoral scholar at Massachusetts Institute of Technology, an assistant professor in University of Minnesota, and an assistant followed by associate professor in University of Massachusetts Amherst. Arya is a recipient of a Distinguished Dissertation Award for his Ph.D. thesis, the NSF CAREER award, an EURASIP Best Paper Award, and the ISIT Jack K. Wolf Student Paper Award. He is also a Distinguished Lecturer of the IEEE Information Theory Society, 2023-24. He is currently serving as an Associate Editor for the IEEE Transactions on Information Theory and as an Area editor for Now Publishers Foundation and Trends in Communication and Information Theory. Arya’s research interests include information theory, coding theory, statistical learning and optimization.
- Mentoring Style: Plan to involve my PhD students/postdocs this time
- Suggested Prerequisites: Some course on Machine Learning
- Summer Tasks: Look up quantization in deep learning
- Previous Project
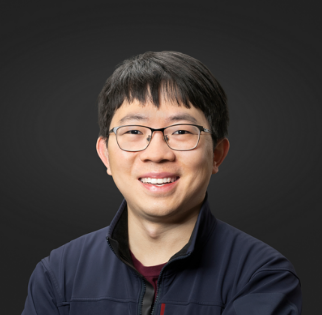
Developing Open Datasets, Models, Systems, and Evaluation Tools for Large (Language) Models
Hao Zhang • haz094@ucsd.edu
B04 6 seats Monday or Wednesday Afternoon, In-Person
The rapid advancement of large multimodal models has revolutionized AI systems, resulting in unprecedented levels of intelligence as seen in OpenAI’s GPT-4. However, despite its performance, the training and architecture details of GPT-4 remain unclear, hindering research and open-source innovation in this field. In this project, we'll explore three relevant areas to LLMs:
- On the system side: infrastructure for scalable training and high-throughput serving with advanced memory management and parallelization techniques.
- On the model side, build multimodal model close to ChatGPT quality, which can also interact with the real world by taking actions and using tools.
- On the data and benchmark side, develop a highly curated data sets and benchmark platform with novel data augmentation, data filtering, and ranking methods.
Read more
- About: Hao Zhang is an Assistant Professor in Halıcıoğlu Data Science Institute and the Department of Computer Science and Engineering at UC San Diego. Before joining UCSD, Hao was a postdoctoral researcher at UC Berkeley working with Ion Stoica (2021 - 2023). Hao completed his Ph.D. in Computer Science at Carnegie Mellon University with Eric Xing (2014 - 2020). During PhD, Hao took on leave and worked for the ML platform startup Petuum Inc (2016 - 2021). Hao's research interest is in the intersection area of machine learning and systems. Hao's past work includes Vicuna, FastChat, Alpa, vLLM, Poseidon, Petuum. Hao’s research has been recognized with the Jay Lepreau best paper award at OSDI’21 and an NVIDIA pioneer research award at NeurIPS’17. Hao also cofounded the company LMNet.ai (2023) which has joined Snowflake since November 2023, and the nonprofit LMSYS Org (2023) which maintains many popular open models, evaluation, and systems.
- Mentoring Style: I am hands-off. I will ask my students to help on some coding details.
- Suggested Prerequisites: DSC102, DSC 140A
- Summer Tasks: Reading LLM papers, get familiar with tools like huggingface, pytorch, FSDP, Megatron-LM, Deepspeed.
- Previous Project
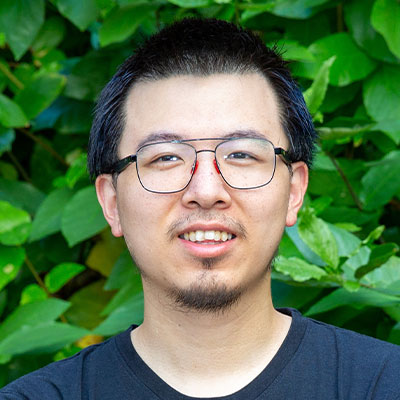
LLM-Based Applications
Jingbo Shang • jshang@ucsd.edu
B05 4 seats Wednesday 11AM-12PM, Zoom
We are at the LLM era. How to leverage LLMs to develop new apps is a fundamental direction. We will talk about some history and state-of-the-art language models, and also learn to use the LLM APIs. Finally, we will brainstorm the LLM-based application ideas and develop cool demo systems.
Read more
- About: I’m an Assistant Professor at UCSD jointly appointed by Computer Science and Halıcıoğlu Data Science Institute. I obtained my Ph.D. from UIUC advised by Prof. Jiawei Han in 2019. I received my B.E. from SJTU in 2014. I’m also a coach of the UCSD’s ACM-ICPC team.
- Mentoring Style: Just me + capstone students. Brainstorming + advice.
- Suggested Prerequisites: DSC 148 required. NLP courses recommended.
- Summer Tasks: Some frontend experiences + ChatGPT use experience.
- Previous Project
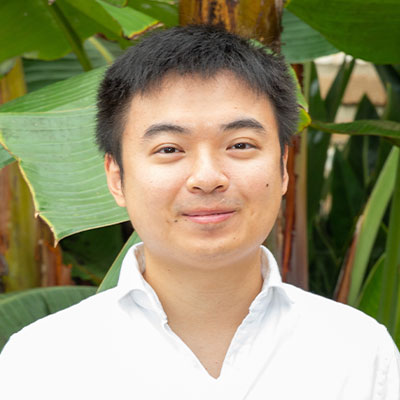
Large Language (Multi-Modal) Model Reasoning
Zhiting Hu • zhh019@ucsd.edu
B06 6 seats Tuesday 3-4PM, In-Person
A central topic in Large Language Model (LLM) research is to enhance their ability of complex reasoning on diverse problems (e.g., logical reasoning, mathematical derivations, and embodied planning). Rich research has been done to generate multi-step reasoning chains with LLMs, such as Chain-of-Thoughts (CoT), Tree-of-Thoughts (ToT), Reasoning-via-Planning (RAP), among others. This capstone aims to explore the diverse reasoning approaches of LLMs (and/or large multi-modal models) and investigate improvement, applications, and scalable implementations of these approaches. For example: (1) Proposing new reasoning algorithms or improvement over existing reasoning algorithms in terms of performance; (2) Developing algorithmic and/or system innovations to scale up existing advanced reasoning algorithms; (3) Applying the reasoning algorithms on new applications in various domains (e.g., chemistry, physics, medicine).
Read more
- About: Zhiting Hu is an Assistant Professor in Halicioglu Data Science Institute at UC San Diego. He received his Bachelor's degree in Computer Science from Peking University in 2014, and his Ph.D. in Machine Learning from Carnegie Mellon University in 2020. His research interests lie in the broad area of machine learning, artificial intelligence, natural language processing, and ML systems. In particular, he is interested in principles, methodologies, and systems of training AI agents with all types of experience (data, symbolic knowledge, rewards, adversaries, lifelong interplay, etc), and their applications in controllable text generation, healthcare, and other application domains. His research was recognized with best demo nomination at ACL2019 and outstanding paper award at ACL2016.
- Mentoring Style: Students can either join the mentor's research group to work closely with PhD students/postdocs on relevant projects, or propose their own ideas and lead the projects. Students are expected to be independent, and mentor will provide necessary advices if needed (PhD students/postdocs can also provide more hands-on guidances).
- Suggested Prerequisites: Large language models; open-source tools such as huggingface
- Summer Tasks: [1] https://arxiv.org/abs/2404.05221; [2] https://arxiv.org/abs/2305.14992
- Previous Project
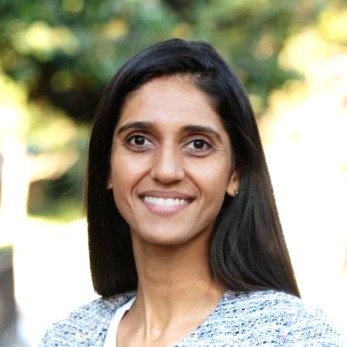
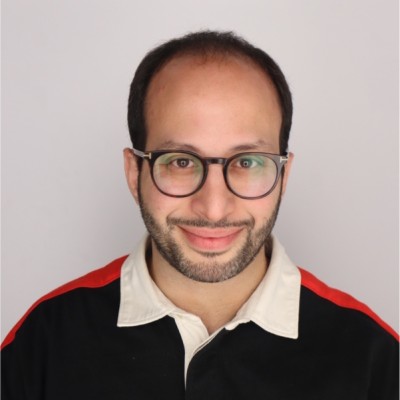
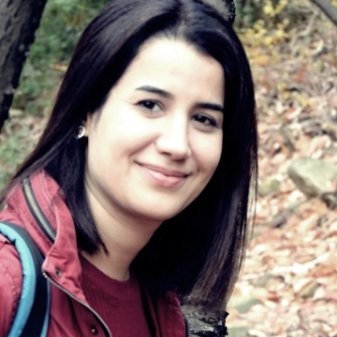
Guardians 🦹‍♀️ of the Generative Realm 👾: Implementing LLM Guardrails
Nimu Sidhu, Abed El-Husseini, and Somayeh Koohbor • With questions for industry partners, email Suraj
and
B07 8 seats Tuesday 12-1PM, Zoom
Industry Partner: Deloitte
This class is for students interested in learning more about large language models and how to make them safe, secure, and private using robust guardrails.
In the first quarter we will:
- Briefly review the LLMs, benchmarking tools, and common enterprise applications
- Discuss when GenAI breaks down and what we can do to mitigate these breakdowns
- Review the most popular frameworks (Guardrails AI, NeMo, and LLM Guard) and architectures leveraged for guardrail implementations
- Implement an enterprise application using one of the popular frameworks introduced
By the end of the course, students can expect to implement a guardrail framework of their choice for their own GenAI application.
Read more
- About: Nimu - Nimu is an AI Solution Architect at Deloitte’s US Delivery Center. Experienced in developing AI courses and delivering large-scale client solutions, she is excited to teach this class. Based in Washington DC 🏛️🌸, Nimu serves as Vice President for the local Returned Peace Corps 🕊 chapter. You can also find her enjoying DC's cultural scene or nurturing her rooftop garden️.
Abed - Abed is a Data Science Manager focused on GenAI applications at Deloitte Consulting. He loves teaching and has previously served as a business case mentor for the HDSI – Deloitte Business Case program. Abed is a graduate of THE Ohio State University and lives in the capital of live music Austin, Texas 🤠🎸 with his wife and son. He’s an avid runner and loves dessert, in that order.
Somayeh - Somayeh is a senior Data Scientist in Deloitte US consulting department. She is an applied scientist with over 10 years of academic and industry driven research involving Data Science, AI and Machine Learning. Somayeh can utilize the latest research, state of the art algorithms, and machine learning techniques to translate data into key strategic insights and actions. - Mentoring Style: Casual, engaging, fun
- Suggested Prerequisites: 1. NLP background (required)
2. Basic LLM implementation (required)
3. Vector store familiarity (nice-to-have)
4. Streamlit/Gradio/Flask experience (python back-end frameworks for web applications)
As part of summer tasks, students can shore up on these skills. - Summer Tasks: Research one implementation for any LLM guardrail and develop a 5 min presentation. Presentations will take place across the first four class sessions.
Examples to consider:
- From scratch implementation (OpenAI Cookbook)
- LLM Guard
- Guardrails AI
- NVIDIA NeMo Guardrails
- Tru Lens
- Agent-based modeling
Please also shore up on the pre-requisites.
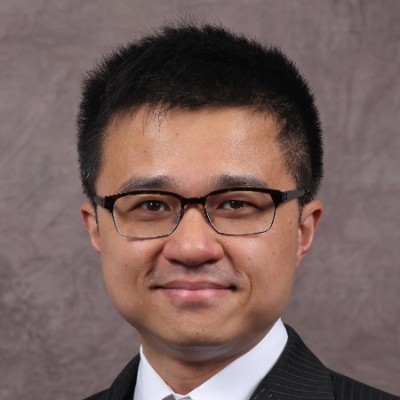
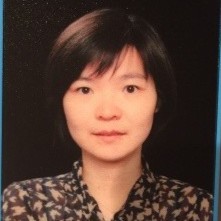
Seeking Alpha in the Sea of Data
Hungjen Wang and Rinne Han • With questions for industry partners, email Suraj
B08 6 seats Friday 10-11AM, Zoom
Industry Partner: Franklin Templeton
This project aims to critically assess the predictive accuracy of financial analysts with respect to the companies they cover. It focuses on understanding the correlation between analysts' forecasts and actual market performance. Another project is about using unstructured data to predict asset returns. This project explores the innovative application of Large Language Models (LLMs) in forecasting asset returns, particularly focusing on the integration of unstructured data with traditional time-series data.
Read more
- About: Hi Everyone, I am Hungjen. I currently work in Franklin Templeton as Head of AI and Optimization Research. I have worked in the Tech and Financial industry for over a decade. We are currently working on build Gen AI systems to enhance the efficiency and accuracy of the workflow. It would be great to participate in this program and hear your new ideas.
Hello everyone, I am Rinne. I am the Lead of Reach Scientist in AI & Optimization Research Team in Franklin Templeton and working on Financial GenAI applications. Welcome to pronounce my name as Renee. I have over 12 years of business experiences on Machine Learning model based applications across different business domains i.e. retail content/image recommendation, dynamic pricing, ads campaign optimization, forecasting, marketing attribution and CRM. I got a Master and a PhD degree from Nagoya University in Japan at 2005 and 2011 where I had dived deep into research areas in statistical learning, data mining and machine learning. My current research interests cover GenAI, reinforcement learning, graphic modeling, recommendation, forecasting and machine learning in Finance etc. Outside of work, I like to be with my family to read books, enjoy yoga, outdoor activities and volunteer activities. - Mentoring Style: We will form a small team and laser focus on a well defined problem to solve.
- Suggested Prerequisites: Advanced programming skills
- Summer Tasks: LangChain, PyTorch
⚙️ Applied Data Science
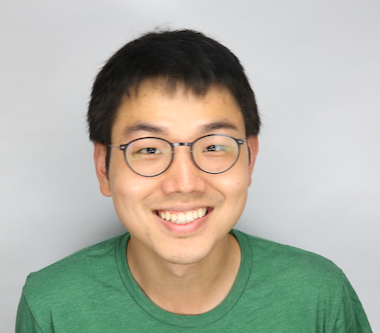
Low Cost Privacy Engineering
Haojian Jin • h7jin@ucsd.edu
B09 6 seats Monday Afternoon, In-Person
This group is for students interested in Human-Computer Interaction, Software Engineering, AI, Mobile Computing, Programming Language, and Cyber-physical Systems. Privacy engineering is expensive for startups. We will explore new techniques that can lower the cost of privacy engineering. Through the project, students will learn human-centered system design.
Read more
- About: Our lab, Data Smith Lab, studies the privacy and security of data systems by researching the people who design, implement, and use these systems.
- Mentoring Style: Weekly meetings. I will offer pointers and feedback.
- Suggested Prerequisites: DSC 102
- Summer Tasks: Learn Django and React.
- Previous Project
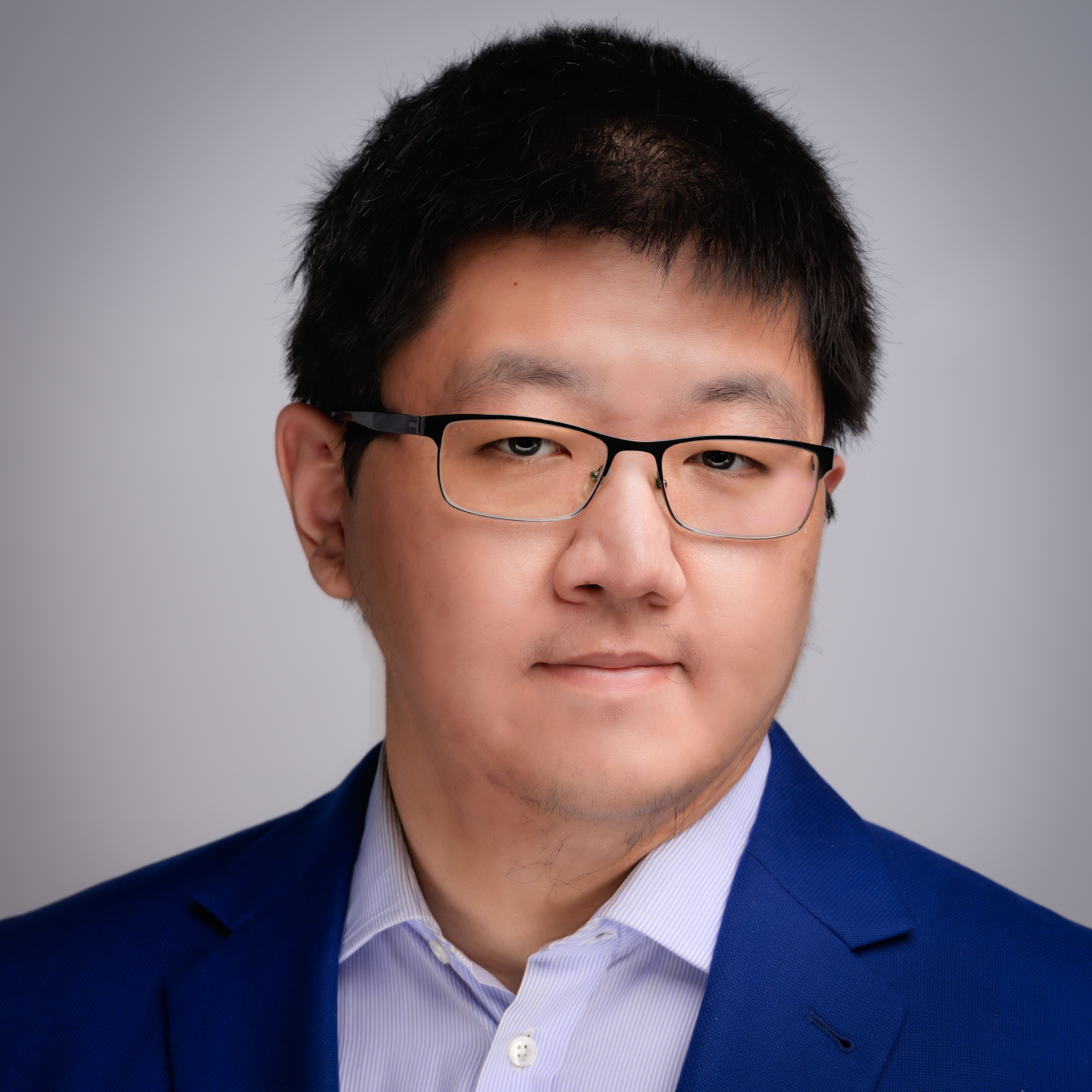
Hunting for Ghost Particles: Analyzing Time Series Data produced by Semiconductor Detectors
Aobo Li • aol002@ucsd.edu
B10 6 seats Monday 3-4PM, In-Person
Neutrinos are tiny particles that are almost like ghosts because they can pass through just about anything without being noticed. They're produced in huge numbers by the sun and other stars, but catching them is really tough because they hardly ever interact with other matters. Scientists use special, super sensitive equipment such as Semiconductor Detector to try and spot these sneaky particles and learn more about how the universe works. The Majorana Demonstrator experiment utilizes an array of these Semiconductor Detectors to capture neutrinos hidden in the time series data generated by these detectors. In this project, we will establish an analysis team dedicated to examining this time series data. The team will undertake multiple analytical tasks, including employing machine learning models for time series classification and regression, aiming to produce an energy spectrum akin to the one generated by the Majorana Demonstrator.
Read more
- About: I am Aobo Li (you can call me obo, like the musical instrument). I am a new faculty at HDSI & the Department of Physics. I earned my B.S. from UW Seattle and my PhD from Boston University, both in the field of physics. My research uses machine learning to squeeze out the maximum amount of information from ultra-sensitive radiation detectors, all in the quest to uncover extremely rare physics events in our universe. Beyond academia, my interests span from following e-sports to exploring national parks and photography.
- Mentoring Style: To achieve our final analysis goal—the detector spectrum—students will need to construct and train 3-5 machine learning models using a fully labeled dataset. One of these models will address a regression task, while the others will tackle binary classification, using 0/1 labels. An Analysis Coordinator (AC) will oversee the entire model-building process and document everything in a unified analysis document. Within the project, we will form subgroups; each will select a machine learning task, propose a model to accomplish it, and provide weekly updates during meetings to track progress. The AC and I will engage with each student weekly to discuss their tasks and provide feedback on their updates. Additionally, students will receive detailed assistance from the AC on coding and technical aspects, whereas I will focus on providing in-depth guidance to the AC.
- Suggested Prerequisites: None
- Summer Tasks: Data Prerequisite:
The Majorana Demonstrator data we will analyze is already available online:
Data Download Website: https://zenodo.org/records/8257027
Data Release Notes: https://arxiv.org/pdf/2308.10856
All students who wish to get involved in this project should make sure to read the Data Release Notes carefully. Students should also try to download the data and make sure they can extract informations from it (the data is stored in .hdf5 file format).
Machine Learning Prerequisite:
Students should make sure they can design, run and validate machine learning models for classification and regression tasks, ideally using PyTorch to build and train simple neural networks. During the data analysis process, student will have the freedom to pick their own models to use.
Analysis Coordinator:
One of the enrolled student will be elected as the analysis coordinator (AC) of this project. AC does not have to build a machine learning models on their own, but they will need to coordinate model development among different subgroups and manage this project at a higher level. This will be an excellent leadership experience that can be highlighted on student's CV. If you are interested in this position, please send an email to aol002@ucsd.edu.
Additional reading:
Nachman Undergraduate Thesis: https://drive.google.com/file/d/1oF8oiGke5SCVbKTbbPlNwxh9zYN_Nri4/view?usp=sharing Please pay special attention to Section 3: Pulse Shape Parameter Pipeline
Majorana Demonstrator Experiment: https://phys.org/news/2023-02-legacy-majorana.html
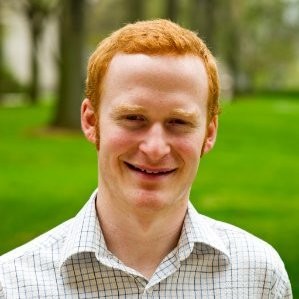
Learning How to Make a Better Solar Cell using Molecular Graphs
David Fenning • dfenning@ucsd.edu
B11 4 seats Thursday 2-3PM, In-Person
Perovskite solar cells are an emerging technology that holds promise to revolutionize the PV industry given their unprecedented performance. Small molecules are added to these solar cells to terminate chemical bonds at the interfaces of the perovskite to enable improved stability. Today, the discovery of such molecules is done largely by Edisonian experimentation. A significant challenge is the broad chemical space, and the complexity of of the interface limits the application of theory. We seek to use literature mining and complimentary automated experiments in our lab run by python scripts to learn what makes molecules successful using graph-based representations of the molecules and to optimize the graphs to discover new molecules and gain deeper insight into the problem.
Read more
- About: I'm a materials scientist who is working on developing platforms for accelerated discovery of new solar energy conversion materials.
- Mentoring Style: Inclusion of PhD students in the meetings working in the materials science domain and a staff research associate working on coding scripts for experiments and ML on our database. The discussions will be cross-disciplinary with all of us learning together to solve new problems.
- Suggested Prerequisites: None
- Summer Tasks: nan
- Previous Project
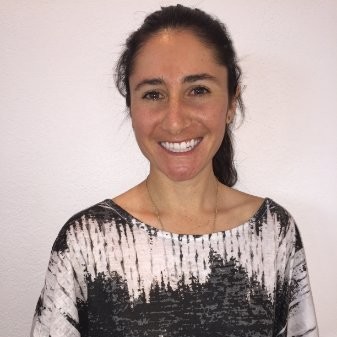
Graph ML for Chip Profiling
Lindsey Kostas • With questions for industry partners, email Suraj
B12 10 seats Monday 1-2PM, In-Person
Industry Partner: Qualcomm
Machine Learning is becoming an increasingly necessary technique in the design of chips due to the end of Moore’s Law and the increased complexity of the process, functionality requirements, and design time limits. A circuit represents a complex graph with unique properties that do not exist in more common graph ML applications such as those for social networks or biologic entities. As a result, graph machine learning offers a powerful set of techniques to understand the fundamental properties of the chip design and thereby create better designs more quickly. This capstone will expose students to graph algorithms and graph ML through the exploration of unsupervised learning on chip designs and equip them with the skills to tackle arbitrary graph modeling tasks.
Learning Objectives:
- Develop deep understanding of graph analysis techniques, both classical graph algorithms and machine learning approaches.
- Gain exposure to a variety of Graph ML architectures and their properties.
- Develop an intuition for selection of graph modeling architectures based on the characteristics of the underlying graph of interest.
- Explore custom architectures to handle complex graph structures.
- In the absence of ideal labels, learn how to develop and unsupervised ML solution or define proxy tasks for training a model with the desired properties.
- Learn the basics about chip design and ML for chip design.
Read more
- About: Lindsey is a Senior Staff Machine Learning Engineer. She joined a nascent ML R&D team at Qualcomm in 2018 and since that time she has led multiple projects in ML-based CAD/EDA which have impacted global SoC design process for teams across the globe leading to significant savings in time-to-market, compute and NRE cost. She holds two granted and five pending patents related to this work and consults on a variety of ML-driven initiatives across the company in application ranging from digital and analog design to 5G to licensing. In 2021, she was honored by the Global Semiconductor Association (GSA) as the inaugural Female Up-And-Comer for her exceptional contributions toward the development, innovation, growth, and success in the semiconductor industry.
Prior to joining Qualcomm, Lindsey was a 4-year scholarship athlete at Stanford University where she won two tennis national team championships and was honored as an Elite 89 Award Finalist. After graduating with distinction in Economics, she obtained her master’s degree in Computer Science with an emphasis in Artificial Intelligence from Stanford University. While in the master’s program Lindsey was a teaching assistant and a research associate for Chris Re and Jure Leskovic with an emphasis in deep representation learning. Her current research interests are building interpretable and explainable optimization solutions which combine traditional ML, generative AI, and classical algorithms and how to translate ML solutions into usable end-to-end tools. - Mentoring Style: Mentor team will be hands on, available for discussion outside of class and office hours. We will bring guest speakers/advisors as relevant.
- Suggested Prerequisites: Students will be most successful if they have experience with deep learning, graph algorithms/ML, data analysis techniques. Students will also benefit if they have a background or interest in chip/circuit design.
- Summer Tasks: Go through the course at: https://web.stanford.edu/class/cs224w/
Be familiar with pytorch, pytorch geometric, network x - Previous Project
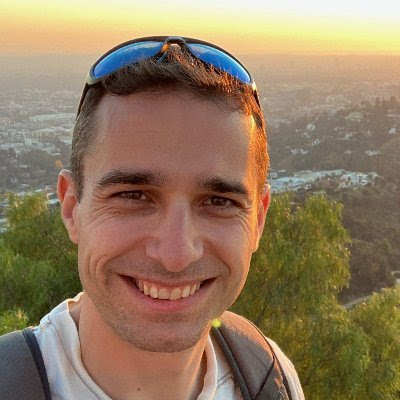
Deep Learning for Climate Model Emulation
Duncan Watson-Parris • dwatsonparris@ucsd.edu
B13 6 seats Wednesday 2-3PM, In-Person
The choices humanity makes in the next few decades will determine how much warmer the Earth will be by the end of the century, with implications for billions of lives and trillions of dollars in GDP. Many different emission pathways exist that are compatible with the Paris climate agreement, and many more are possible that miss that target. While some of the most complex climate models have simulated a small selection of these, it is impractical to use these computationally expensive models to fully explore the space of possibilities or assess all the associated risks. Our lab has recently developed state-of-the-art climate model emulators to enable fast, accurate and reliable predictions for any given scenario (https://github.com/duncanwp/ClimateBench). This project will extend this work by incorporating multiple climate models at different levels of fidelity to provide high-resolution predictions with robust uncertainties for improved decision making.
Read more
- About: Duncan Watson-Parris is an atmospheric physicist working at the interface of climate research and machine learning to investigate the effect of air-pollution on the climate. Using cutting-edge machine learning techniques to combine global models with satellite data his group looks to better understand complex aerosol-climate interactions and improve projections of climate change. He recently moved to San Diego from Oxford, England and enjoys soccer, chess and role-play games but is currently learning to surf!
- Mentoring Style: This work is central to my research interests and will be integrated into my broader group program to the extent the students want to engage with it. The students will be welcome to join my group meetings (typically held at Scripps Institution of Oceanography).
- Suggested Prerequisites: DSC 140A
- Summer Tasks:
- Skim the latest UN Intergovernmental Panel on Climate Change Synthesis Report to get a summary of the latest climate change science, especially the figures: https://www.ipcc.ch/report/ar6/syr/downloads/report/IPCC_AR6_SYR_SPM.pdf
- Read the ClimateBench paper: https://agupubs.onlinelibrary.wiley.com/doi/full/10.1029/2021MS002954
- Try out the xarray Python library for working with climate data: https://docs.xarray.dev/en/stable/
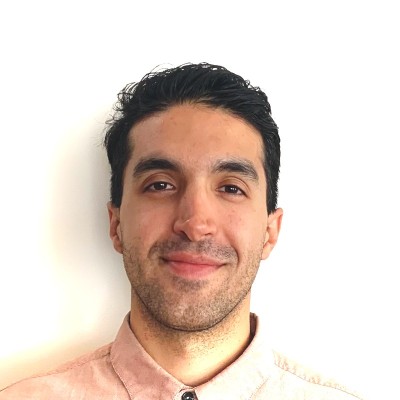
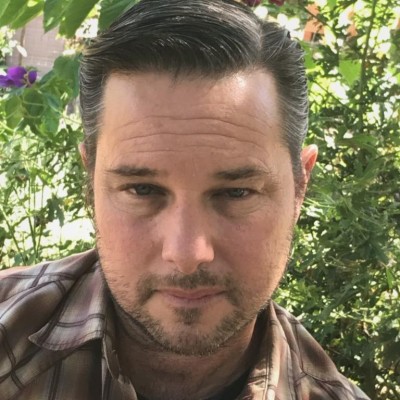
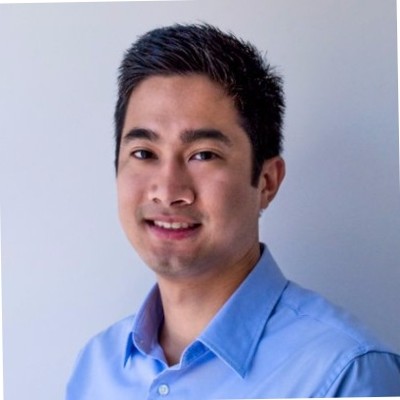
Igniting Resilience: Unleashing Wildfire Mitigation Insights
Kasra Mohammadi, Robert D. Flamenbaum, and Phi Nguyen • With questions for industry partners, email Suraj
and
B14 8 seats TBD (Schedule with mentor), Hybrid
Industry Partner: SDG&E
In partnership with SDG&E's Wildfire Mitigation Team, students will embark on a pivotal journey to propel wildfire mitigation risk assessment forward by investigating the intricate connections between electrical assets, urban infrastructure, and environmental factors. This venture is pivotal for fostering a risk-informed and smarter tomorrow. By diving into real-world data from diverse sources such as snapshot-specific asset attribute data, ignition and outage event data, geospatial and environmental datasets, participants will utilize a suite of analytical tools including predictive modeling, graph theory, and time-series analysis to unearth insights. The endeavor will harness these analyses to pinpoint locations and assets that pose the highest risk of wildfire and relative and absolute severity of that risk. Additionally, projects will leverage predictive modeling techniques to forecast and prepare for future states of the electric system and the environment where change is the norm. Through this hands-on experience, students will not only contribute to San Diego's wildfire mitigation strategies but also develop valuable skills that echo the needs of a world class utility grid.
Read more
- About: Kasra Mohammadi is a Data Scientist at San Diego Gas & Electric, working under the Risk Analytics team within the Wildfire Mitigation department, helping lead the company forward in its wildfire mitigation initiatives. Kasra is a fellow UCSD alum, having graduated from UCSD with bachelor’s degree in electrical engineering and went on to earn a master’s degree in data Analytics from Clarkson University. He has worked for several years within the wildfire mitigation and utility space where his focus has been on developing, optimizing, and managing various wildfire risk assessment and mitigation models and tools. Kasra joined SDG&E to focus on developing models to mitigate wildfire risk in California and has since continued to push the efficiency and quality of those models to help benefit the Wildfire Mitigation efforts pursued by SDG&E.
Robert D. Flamenbaum is an accomplished data scientist and team leader specializing in wildfire mitigation risk analytics at San Diego Gas & Electric. He boasts a strong educational background with a Master of Science in Data Science from Southern Methodist University and multiple professional certificates, including Database Administration Using Oracle and Geographic Information Systems. Robert has led significant projects, such as the development of the WiNGS model and SDG&E's Electric Distribution Engineering analytics roadmap. His expertise encompasses machine learning, Python programming, GIS web development, and electric distribution asset failure prediction. Recognized for his contributions, Robert has received accolades such as the Bertha Lamme Top Innovator Award and multiple awards at ESRI International User Conferences.
Dr. Phi Nguyen is a senior data scientist at San Diego Gas & Electric, where he leads the Data Science Center of Excellence. Dr. Nguyen graduated from UCSD with a Ph. D. in materials science and engineering, where he developed nanomaterials for clean energy applications. He has worked for several years as a consultant in the energy sector, where his focus was on using data to support policies that promote clean energy and energy efficiency. Dr. Nguyen joined SDG&E to focus on developing models to mitigate wildfire risk in California and has since expanded his work to other areas that benefit San Diego communities. - Mentoring Style: The student group will be a stand-alone unit at SDG&E led by Mentors. Mentors will first work with students to understand utility space, and then schedule time with other SDG&E staff who will provide tours, field visits, and other utility-specific training. Students will also be introduced to other data scientists and engineers at SDG&E who are available for support on an as-needed basis throughout the duration of the project. However, once an introduction is made, it will be up to the students to reach out to staff when support is needed. Students will be encouraged to present their ideas by staff members beyond the mentors.
- Suggested Prerequisites: None
- Summer Tasks:
- Previous Project
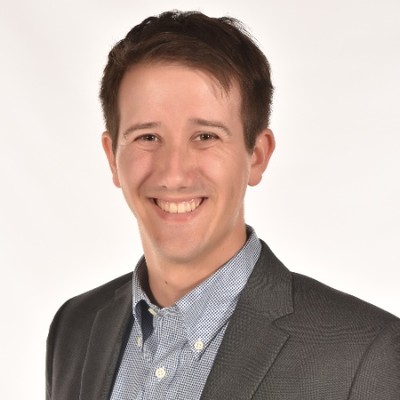
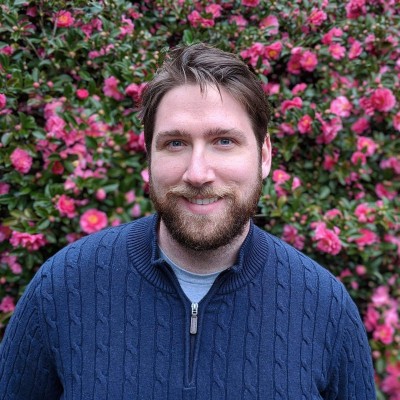
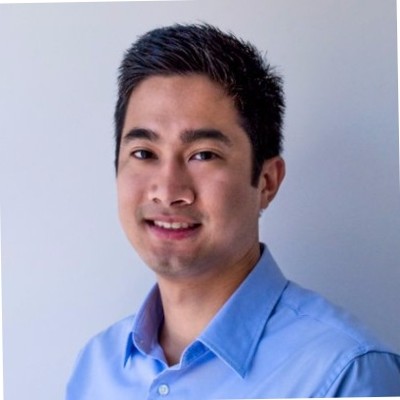
Powering Progress: Crafting Reliable EV Infrastructure in San Diego
Ari Gaffen, James McCloskey, and Phi Nguyen • With questions for industry partners, email Suraj
and
B15 8 seats TBD (Schedule with mentor), Hybrid
Industry Partner: SDG&E
In partnership with SDG&E's Clean Transportation Team, students will embark on a pivotal journey to propel electric vehicle (EV) adoption forward by investigating the intricate connections between EVs, urban infrastructure, and energy dynamics. This venture is pivotal for fostering a sustainable tomorrow. By diving into real-world data from diverse sources such as historical EV adoption statistics, community engagement on EV platforms, and the extensive road networks from OpenStreetMap, participants will utilize a suite of analytical tools including time-series analysis, graph theory, and text analytics to unearth insights. The endeavor will harness these analyses to pinpoint strategic locations for EV charging stations, aiming to build a robust and accessible infrastructure. Additionally, projects will leverage predictive modeling techniques to forecast and prepare for the evolving demands of a future where electric mobility is the norm. Through this hands-on experience, students will not only contribute to San Diego's transition to clean transportation but also develop valuable skills that echo the needs of an eco-conscious society.
Read more
- About: Ari Gaffen is a Principal Data Analyst at San Diego Gas & Electric, working under the Data Analytics and Reporting team within the Clean Transportation department. Ari graduated from UCSD with bachelor’s degree in math and economics and went on to earn a master’s degree in applied economics from San Diego State University. During his tenure at SDG&E, Ari has focused on compliance reporting, internal analytics, and creating efficiencies using scripting languages and ETL jobs. In addition to working at SDG&E, Ari has also been an adjunct professor at SDSU where he taught an upper division Excel class for marketing majors. Ari joined SDG&E to help increase the internal efficiency of the Billing departments operations and has since expanded his work to other areas that benefit Clean Transportation Programs.
James McCloskey is a Project Manager at San Diego Gas & Electric, where he leads IT and non-Infrastructure Projects for the Clean Transportation Department. James graduated from UCSD with bachelor’s degree in cognitive science and went on to earn a master’s degree in manufacturing systems engineering from Cal State Northridge. He has worked for several years in the energy sector where his focus has been on building EV Charging Infrastructure Systems. James joined SDG&E to focus on building out dynamic hourly rates in the SDGE billing system and has since expanded his work to other areas that benefit Clean Transportation Programs.
Dr. Phi Nguyen is a senior data scientist at San Diego Gas & Electric, where he leads the Data Science Center of Excellence. Dr. Nguyen graduated from UCSD with a Ph. D. in materials science and engineering, where he developed nanomaterials for clean energy applications. He has worked for several years as a consultant in the energy sector, where his focus was on using data to support policies that promote clean energy and energy efficiency. Dr. Nguyen joined SDG&E to focus on developing models to mitigate wildfire risk in California and has since expanded his work to other areas that benefit San Diego communities. - Mentoring Style: The student group will be a stand-alone unit at SDG&E led by Mentors. Mentors will first work with students to understand utility space, and then schedule time with other SDG&E staff who will provide tours, field visits, and other utility-specific training. Students will also be introduced to other data scientists and engineers at SDG&E who are available for support on an as-needed basis throughout the duration of the project. However, once an introduction is made, it will be up to the students to reach out to staff when support is needed. Students will be encouraged to present their ideas by staff members beyond the mentors.
- Suggested Prerequisites: None
- Summer Tasks:
- Previous Project
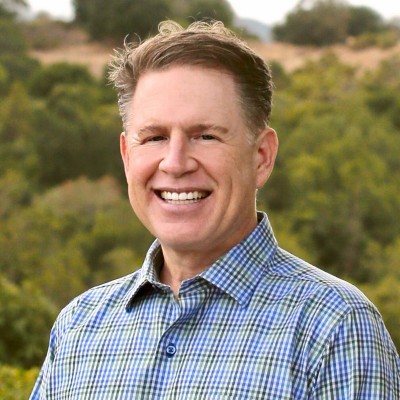
Blockchain
Sheffield Nolan • With questions for industry mentors, email Suraj
B16 8 seats Wednesday 2-3PM, Zoom
Industry Partner: Franklin Templeton
The project domain for college students pursuing degrees in Data Science within the blockchain field presents an exciting and dynamic landscape for exploration and innovation. In this domain, students can engage in projects that involve leveraging blockchain technology to address various real-world challenges. They can design and develop smart contracts for applications such as supply chain management, digital identity verification, or decentralized finance (DeFi). Students can also explore the integration of blockchain with emerging technologies like Internet of Things (IoT) or Artificial Intelligence (AI), enabling secure and transparent data exchange and enhancing data privacy. Furthermore, they can analyze blockchain data to identify patterns, detect anomalies, and develop predictive models for optimization and decision-making. By engaging in such projects, students gain practical experience in blockchain development, data analysis, and problem-solving, enabling them to contribute to the advancement of this transformative technology and become key players in the blockchain ecosystem.
Read more
- About: Sheffield Nolan is an enterprise architect for Franklin Templeton focusing on FinTech innovation. Sheffield advises and provides technical guidance for early stage fintech companies within Franklin Templeton’s fintech partnerships and corporate strategic investments.
Sheffield specializes in many key areas of FinTech including Artificial intelligence (AI) and Blockchain with an emphasis on DeFi, zero knowledge proofs, generative adversarial networks, Bidirectional Encoder Representations from Transformers (BERT) and Generative Pre-trained Transformers (GPT)
He is also a contributor to the official Coinbase Python API project on Github.
Prior to joining Franklin Templeton, Sheffield was the founder and CEO/CTO of AppRedeem, an innovator in the mobile rewards space. Sheffield led AppRedeem's venture funding through 2 rounds totaling $1.7MM from Blue Run Ventures and SV Angel. AppRedeem was acquired by the publicly traded company Perk in 2015.
Prior to AppRedeem, Sheffield developed apps that climbed to the top 5 paid and free positions in the Apple App Store (U.S. and international markets). Before that he architected and managed large scale solutions for many Fortune 500 companies and venture backed startups, including Visa, eBay and PayPal. - Mentoring Style:
- Suggested Prerequisites: None
- Summer Tasks:
- Previous Project
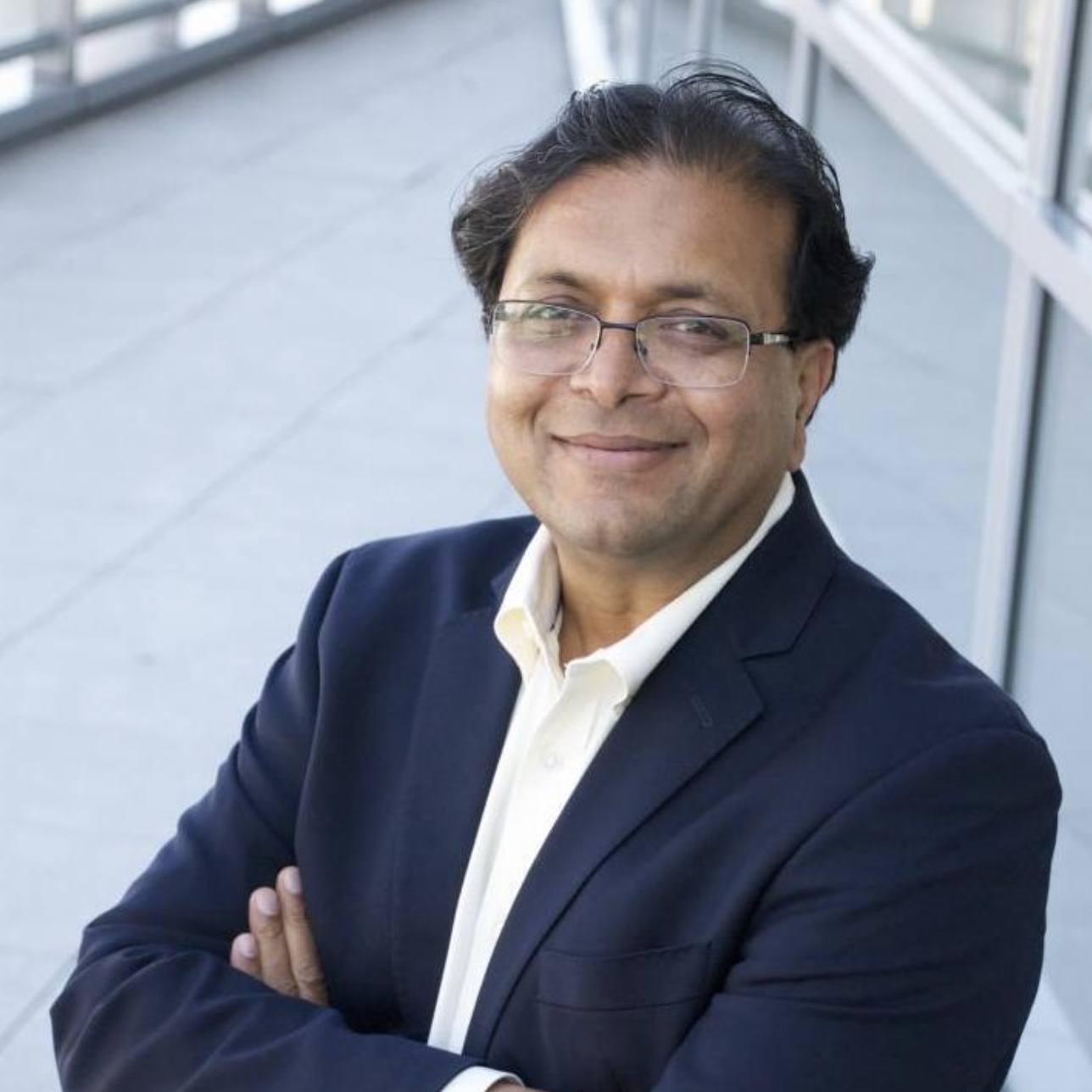
Blockchain
Rajesh Gupta • rgupta@ucsd.edu
B17 4 seats TBD (Schedule with mentor), In-Person
The project will build upon earlier work on GymCoin and Goodwill coins to explore the world of new distributed applications that rely upon Blockchain properties.
Read more
- About: Rajesh Gupta serves as a founding director of the Halıcıoğlu Data Science Institute and as a distinguished professor of Computer Science and Engineering at UC San Diego. His research is in embedded and cyber-physical systems with a focus on sensor data organization and its use in optimization and analytics. Prof. Gupta holds Qualcomm Endowed Chair in Embedded Microsystems at UC San Diego and INRIA International Chair at the French international research institute in Rennes, Bretagne Atlantique. He is a Fellow of the IEEE, the ACM and the American Association for the Advancement of Science (AAAS).
- Mentoring Style: Mostly as a listener to the students.
- Suggested Prerequisites: None
- Summer Tasks:
- Previous Project
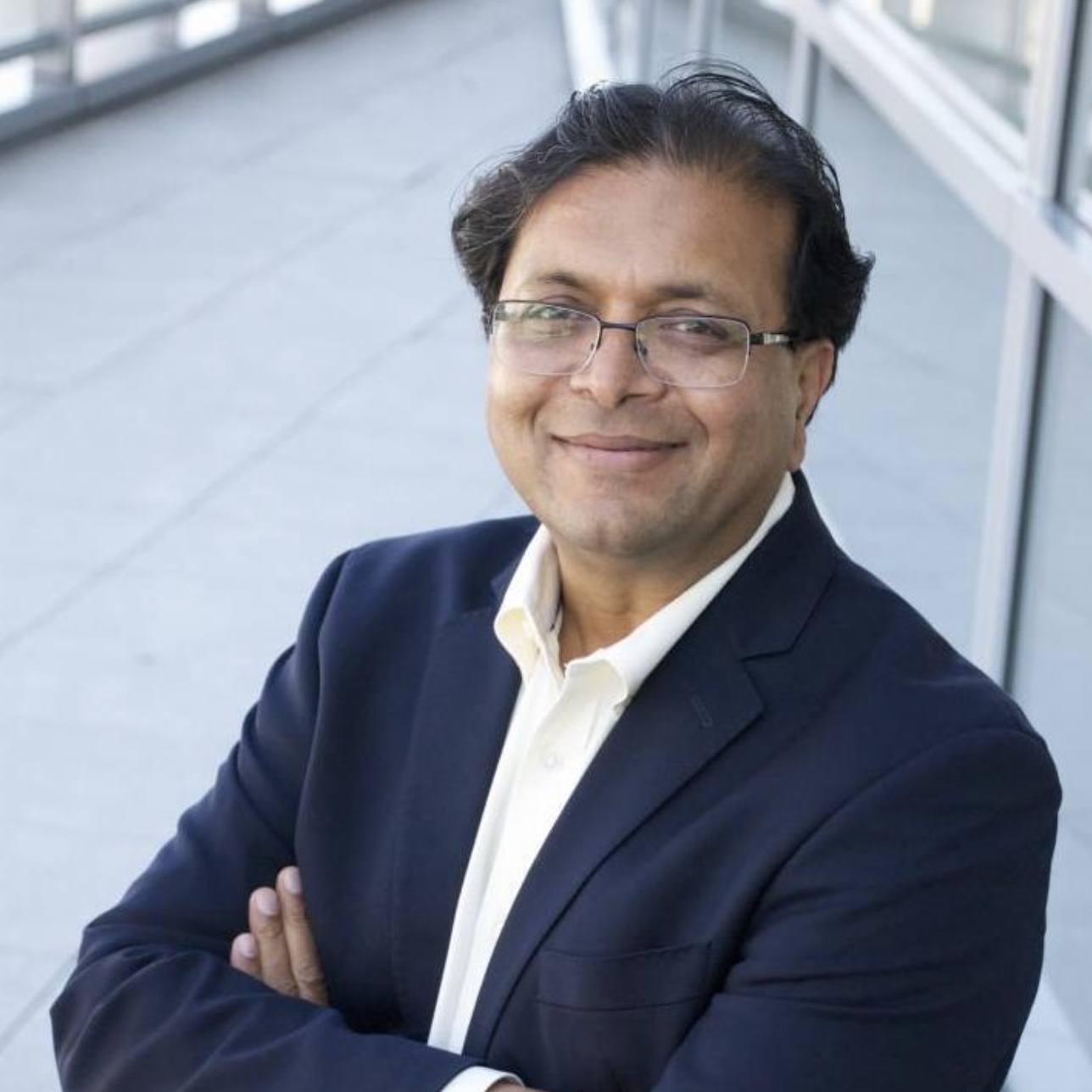
Hardware Acceleration of ML Algorithms
Rajesh Gupta • rgupta@ucsd.edu
B18 4 seats TBD (Schedule with mentor), In-Person
Machine Learning Acceleration using Hardware such as FPGA refers to design and implementation of hardware blocks that are useful in either acceleration of application codes (such as manipulation of graph neural networks) or in acceleration of architectural mechanisms (such as prefetches, memory assists etc). In this project you will explore use of architectural mechanics that substantially speedup the selected ML codes.
Read more
- About: Rajesh Gupta serves as a founding director of the Halıcıoğlu Data Science Institute and as a distinguished professor of Computer Science and Engineering at UC San Diego. His research is in embedded and cyber-physical systems with a focus on sensor data organization and its use in optimization and analytics. Prof. Gupta holds Qualcomm Endowed Chair in Embedded Microsystems at UC San Diego and INRIA International Chair at the French international research institute in Rennes, Bretagne Atlantique. He is a Fellow of the IEEE, the ACM and the American Association for the Advancement of Science (AAAS).
- Mentoring Style: Mostly as a listener to the students.
- Suggested Prerequisites: None
- Summer Tasks:
- Previous Project